Brain Sciences Free Full-Text Heart Rate Variability during Online Video Game Playing in Habitual Gamers: Effects of Internet Addiction Scale Ranking Score and Gaming Performance
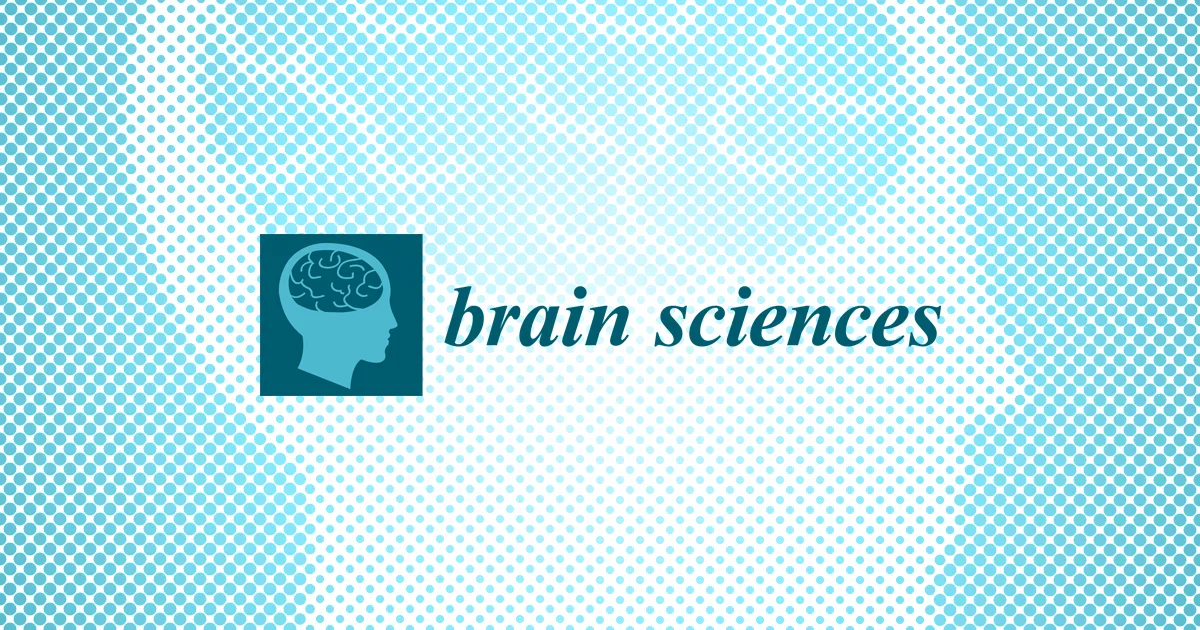
Previous studies have demonstrated that individuals with internet gaming disorder (IGD) display abnormal autonomic activities at rest and during gameplay. Here, we examined whether and how in-game autonomic activity is modulated by human characteristics and behavioral performance of the player. We measured heart rate variability (HRV) in 42 male university student habitual gamers (HGs) when they played a round of League of Legends game online. Short-term HRV indices measured in early, middle and late phases of the game were compared between the players at high risk of developing IGD and those at low risk, as assessed by the revised Chen Internet addiction scale (CIAS-R). Multiple linear regression (MLR) was used to identify significant predictors of HRV measured over the whole gameplay period (WG), among CIAS-R, ranking score, hours of weekly playing and selected in-game performance parameters. The high-risk players showed a significantly higher low-frequency power/high-frequency power ratio (LF/HF) relative to the low-risk players, regardless of game phase. MLR analysis revealed that LF/HF measured in WG was predicted by, and only by, CIAS-R. The HRV indicators of sympathetic activity were found to be predicted only by the number of slain in WG (NSlain), and the indicators of parasympathetic activity were predicted by both CIAS-R and NSlain. Collectively, the results demonstrated that risk of developing IGD is associated with dysregulated autonomic balance during gameplay, and in-game autonomic activities are modulated by complex interactions among personal attributes and in-game behavioral performance of the player, as well as situational factors embedded in game mechanics. clear
You are accessing a machine-readable page. In order to be human-readable, please install an RSS reader.
Find support for a specific problem in the support section of our website.
Get Support Please let us know what you think of our products and services.
Give Feedback Visit our dedicated information section to learn more about MDPI.
Get Information clearby Kehong LongKehong LongSciProfiles Scilit Preprints.org Google Scholar 1,2
, Xuzhe Zhang
Xuzhe ZhangSciProfiles Scilit Preprints.org Google Scholar 1,2, Ningxin Wang
Ningxin WangSciProfiles Scilit Preprints.org Google Scholar 1,3 and Hao Lei
Hao LeiSciProfiles Scilit Preprints.org Google Scholar 1,2,3,*1State Key Laboratory of Magnetic Resonance and Atomic and Molecular Physics, Innovation Academy for Precision Measurement Science and Technology, Chinese Academy of Sciences, Wuhan 430071, China2University of Chinese Academy of Sciences, Beijing 100190, China3Wuhan National Laboratory for Optoelectronics, Huazhong University of Science and Technology, Wuhan 430074, China*Author to whom correspondence should be addressed. Brain Sci.2024, 14(1), 29; https://doi.org/10.3390/brainsci14010029 Submission received: 6 December 2023/ Revised: 14 December 2023/ Accepted: 23 December 2023/ Published: 26 December 2023(This article belongs to the Section Behavioral Neuroscience) Download keyboard_arrow_downDownload PDFDownload PDF with CoverDownload XMLDownload EpubBrowse FiguresReview ReportsVersions Notes
Abstract
:Previous studies have demonstrated that individuals with internet gaming disorder (IGD) display abnormal autonomic activities at rest and during gameplay. Here, we examined whether and how in-game autonomic activity is modulated by human characteristics and behavioral performance of the player. We measured heart rate variability (HRV) in 42 male university student habitual gamers (HGs) when they played a round of League of Legends game online. Short-term HRV indices measured in early, middle and late phases of the game were compared between the players at high risk of developing IGD and those at low risk, as assessed by the revised Chen Internet addiction scale (CIAS-R). Multiple linear regression (MLR) was used to identify significant predictors of HRV measured over the whole gameplay period (WG), among CIAS-R, ranking score, hours of weekly playing and selected in-game performance parameters. The high-risk players showed a significantly higher low-frequency power/high-frequency power ratio (LF/HF) relative to the low-risk players, regardless of game phase. MLR analysis revealed that LF/HF measured in WG was predicted by, and only by, CIAS-R. The HRV indicators of sympathetic activity were found to be predicted only by the number of slain in WG (NSlain), and the indicators of parasympathetic activity were predicted by both CIAS-R and NSlain. Collectively, the results demonstrated that risk of developing IGD is associated with dysregulated autonomic balance during gameplay, and in-game autonomic activities are modulated by complex interactions among personal attributes and in-game behavioral performance of the player, as well as situational factors embedded in game mechanics.Keywords: autonomic nervous system; heart rate variability; internet gaming disorder; multiplayer online battle arena (MOBA) game : Previous studies have demonstrated that individuals with internet gaming disorder (IGD) display abnormal autonomic activities at rest and during gameplay. Here, we examined whether and how in-game autonomic activity is modulated by human characteristics and behavioral performance of the player. We measured heart rate variability (HRV) in 42 male university student habitual gamers (HGs) when they played a round of League of Legends game online. Short-term HRV indices measured in early, middle and late phases of the game were compared between the players at high risk of developing IGD and those at low risk, as assessed by the revised Chen Internet addiction scale (CIAS-R). Multiple linear regression (MLR) was used to identify significant predictors of HRV measured over the whole gameplay period (WG), among CIAS-R, ranking score, hours of weekly playing and selected in-game performance parameters. The high-risk players showed a significantly higher low-frequency power/high-frequency power ratio (LF/HF) relative to the low-risk players, regardless of game phase. MLR analysis revealed that LF/HF measured in WG was predicted by, and only by, CIAS-R. The HRV indicators of sympathetic activity were found to be predicted only by the number of slain in WG (NSlain), and the indicators of parasympathetic activity were predicted by both CIAS-R and NSlain. Collectively, the results demonstrated that risk of developing IGD is associated with dysregulated autonomic balance during gameplay, and in-game autonomic activities are modulated by complex interactions among personal attributes and in-game behavioral performance of the player, as well as situational factors embedded in game mechanics.
1. Introduction
Internet gaming disorder (IGD) has been officially listed as a mental disorder in the 11th revision of the International Classification of Diseases (ICD-11) published by the World Health Organization 1. IGD is characterized by a pattern of persistent or recurrent Internet-based or offline gaming behavior that is manifested by impaired control over the behavior, giving increasing priority to gaming to the extent that it takes precedence over other life interests and daily activities; and continuing or escalating gaming despite its negative consequences 1. Numerous neuroimaging studies have demonstrated that IGD is associated with various abnormalities in the central nervous system (CNS), manifesting as structural plasticity 2,3,4 and altered resting-state functional connectivity in specific brain areas/networks 5,6,7, as well as aberrant functional activations in tasks involving executive control, impulse inhibition and working memory 8,9,10.Abnormal autonomic activities have also been reported in IGD and problematic internet use (PIU) 11,12. PIU has currently no official diagnostic criteria, but can be defined as use of Internet that created psychological, social, school and/or work difficulties in a person’s life 13. The autonomic nervous system (ANS) is coupled to the CNS anatomically and functionally 14. The ANS receives top-down regulation from the CNS 15,16, and provides feedback through the ascending pathways 17,18. The ANS plays an important role in regulating the majority of the body’s internal processes, allowing adaptive responses to internal and external stressors and guaranteeing the body’s homeostatic milieu 14. The dynamic interactions between the CNS and ANS modulate perception, cognition, emotion generation/regulation, memory consolidation and even the sense of self 16,19,20,21,22.Heart rate variability (HRV) is probably the most widely used measurement to assess ANS activity by quantifying the time interval variations between adjacent heart beats 23,24,25. Sympathetic/parasympathetic tones and prevalence are represented by different HRV indices computed in the time, frequency and non-linear domains 26. HRV measurements have been used in laboratory 27,28 and real-world tasks 29,30 to assess ANS activity perturbations associated with cognitive load 31, mental stress 32, emotional arousal 33, executive control 34 and reactivity to reward 35. Moreover, it has been proposed that HRV can serve as an auxiliary diagnostic tool for certain mental diseases 27,36.Compared to none-gamer or occasional/habitual player controls without IGD, players with IGD were reported to have higher heart rates (HRs), lower standard deviation of inter-beat intervals (SDNN), lower root mean square differences between successive inter-beat intervals (RMSSD), as well as lower high-frequency power percentage and higher low-frequency power percentage at rest, indicating a higher sympathetic tone and/or lower parasympathetic/vagal tone in this population 37,38,39,40,41. IGD-related HRV abnormalities at rest were found to correlate with distressed personality total scores 38 and carving ratings 39, and thought to be linked to defective inhibitory control and reduced autonomic flexibility 42,43. A recent meta-analysis concluded that individuals with PIU have significantly different resting state parasympathetic activity from healthy controls 11.Consistent with the observations in those with alcohol addiction and gambling disorder 44,45, individuals with IGD also appeared to have higher HRV reactivity to game cues and during gameplay when compared to individuals without IGD. IGD individuals showed larger changes in low-frequency power/high-frequency power ratio (LF/HF) upon exposure to visual game cues 46. A significant decrease in high-frequency power (HF) during gameplay was observed in IGD individuals, but not in healthy controls 47. When playing a multiplayer online battle arena (MOBA) game, League of Legends (LOL), only individuals with IGD showed significant in-game HF decrease, the amplitude of which correlated negatively with IGD severity assessed by internet addiction test score 48,49.Game content and context are known to affect in-game HRV as well. Playing violent games induced greater changes in HRV compared to playing non-violent games 50,51. Playing a fighting game was associated with lower in-game RMSSD than playing puzzle games, and the players receiving threat appraisal instructions before gameplay showed significantly lower RMSSD than those received challenge appraisal instructions 52. Evidence also suggests that in-game HRV abnormalities observed in IGD individuals were situational, with different manifestations in different periods/phases of the game. For instance, some authors reported that IGD individuals had significant HF decreases in the early phase of the game when gameplay was still relatively easy and not complicated by real-time state of gaming 48,53, while others showed that HF in IGD individuals did not change in the early game phase, but instead decreased significantly when high attention was required, as well as in the last minutes of gameplay when game ending was approaching 49. Like in-game CNS activities, in-game ANS activities/abnormalities observed in these studies might be attributed to dynamic and complicated interactions between human factors of the player and mechanics of the game being played 54,55.Few previous studies have measured in-game HRV in habitual video gamers who are not formally diagnosed with IGD, or examined the factors influencing the in-game HRV in this population. In this study, real-time HR in habitual gamers (HGs) was recorded continuously with a wearable electroencephalogram (EEG) device when playing a round of LOL game under ergonomic and naturalistic conditions. The HR data were analyzed in two different ways. In the first analysis, short-term HRV in three 5-min time windows representing early (EG), middle (MG) and late (LG) phases of gameplay were computed and compared between the individuals at high risk of developing IGD and those at low risk. The aim of the first analysis is to test whether and how in-game HRV is affected by the risk of developing IGD. In the second analysis, multiple linear regression (MLR) was used to identify significant predictors of in-game HRV measured over the whole gameplay period (WG), aiming to test whether in-game HRV and ANS activities are affected by in-game behavioral performance and human characteristics of the player.
2. Materials and Methods
2.1. Participants
Forty-two right-handed male university students having at least 2 years of experience on LOL playing and, at the same time, having played more than 1000 LOL matches in the past were recruited. Written informed consent and demographic information (Table 1) were obtained from each participant before starting the experiments. The risk of developing IGD was assessed using the revised Chen Internet addiction scale (CIAS-R) 56. The skill level of the player in LOL was assessed using the ranking score provided by the official ranking system at the end of last LOL season (i.e., Season 2018). Self-reported hours of weekly LOL playing (HoWP) was used to assess the frequency of LOL playing in the past year. None of the participants had any neurological or psychiatric history, medical history affecting their ANS or sleep deprivation when they participated the study. All participants had normal or corrected normal vision, and were reported to have not used any neurological or psychiatric drugs in the 24 h before the experiment. The research was approved by Human Subjects Institutional Review Board of Wuhan Institute of Physics and Mathematics, Chinese Academy of Science (Protocol Number: WIPM20190303).
2.2. Experimental Procedures
Each participant was asked to play a ranking-mode LOL match on a Dell computer (OptiPlex 3020SSF) with a 23.8-inch screen (DELL E2417H) in a sound-proof room, together with 9 anonymous online players selected automatically by the built-in matchmaking algorithm in the game. A chest strap equipped with a Polar H10 ECG sensor (Polar Electro Oy, Kempele, Finland) was used for HR recording at a sampling rate of 130 Hz 57. The HR data were recorded continuously for 1 min before the game onset and throughout the entire period of gameplay. No bystander was present in the room except the experimenters. Video data of the games were recorded at 15 frames/s using a built-in function provided by LOL.
2.3. HRV Measurement
The HR data were extracted from the Polar Best app (versions 2.3.1) and transformed into R-R intervals. The Kubios HRV Standard freeware (versions 3.3) was used to calculate HRV indices from the R-R interval time series 58. Square root of Baevsky’s stress index (STRESS) 59, three time domain indices (i.e., Mean HR, SDNN and RMSSD) and one nonlinear index (i.e., sample entropy (SampEn)) were calculated, as well as four frequency domain indices calculated with the autoregressive model, including low-frequency power (LF), HF, total power (TP) and LF/HF.
2.4. LOL Game
LOL is one of the most popular MOBA video games worldwide 60. It is known that playing MOBA games is associated with more time spent on video gaming and a higher endorsement of IGD symptoms 61. In each LOL game, 10 players summoned are divided into 2 ad hoc teams. Each player selects and controls his/her own avatar (or champion) to fight with the champions and no-player-controlled characters from the opponent team in a virtual game arena. To win the game, one team needs to destroy the base (or nexus) of the other team while preventing its own base being destroyed. A typical LOL game lasts 20–40 min and ends when the base of one team is destroyed or one team surrenders voluntarily.There is a seven-tier (i.e., Bronze, Silver, Gold, Platinum, Diamond, Master and Challenger) ranking system in LOL (Version 8.22), and within each tier there are five divisions, with five being the lowest in that tier and one being the highest. Game rank of the participants in this study ranged from Bronze to Master, with 5 in Bronze, 18 in Silver, 2 in Platinum, 16 in Diamond and 1 in Master. For statistical analyses, the categorical game ranks of the players were transformed into real number ranking scores using a linear coding system, with 1 representing tier Bronze and 6 representing tier Master. An increment of 0.2 was used to code each within-tier divisional promotion. For example, Division 5 in tier Sliver was coded as 2.0, and Division 3 in tier Diamond as 5.4.A typical LOL game can be divided roughly into EG, MG and LG phases, based on the interim goals and the gaming strategy the player would most likely adopt. Since there are no clear markers that can be used to tell the game phases apart, we defined them operationally. The 5 min immediately after game onset was taken as the EG phase, the 5 min around the midpoint of each game as the MG phase, and the last 5 min of the game as the LG phase. In-game behavioral parameters used for data analysis, including game length, number of slay (Nslay) and number of slain (Nslain), were read directly from the game log.
2.5. Data Analysis
The HRV data were analyzed using two different methods. In the first analysis, the participants were divided into two groups according to their CIAS-R. The participants with a CIAS-R higher than 63 were considered to have a high risk of becoming addicted 56 and therefore assigned into the High-Risk group (n = 20), while those with a CIAS-R lower than 63 were assigned into the Low-Risk group (n = 22) (Table 1). An independent sample t-test or Mann–Whitney U test was used for statistical analysis of the inter-group differences in demographic and in-game behavioral parameters. Repeated measures analysis of covariance (RM-ANCOVA) was used to compare HRV measured in different game phases, with group as the between-subjects factor (High Risk vs. Low Risk), game phase as the within-subjects factor. All demographic parameters listed in Table 1, except CIAS-R, was used as covariates. Independent-sample and paired t-tests were used for post hoc between-subject and within-subject comparisons, respectively. False discovery rate (FDR) correction was applied to control type-I errors associated with multiple post hoc comparisons.In the second analysis, MLR was used to identify significant predictors for in-game HRV measured over WG (27 ± 5 min). All demographic and behavioral parameters listed in Table 1 were included in the regression model, except education and game length. Education had a high correlation with age (r = 0.794, p < 0.001), and game length showed significant correlations with both Nslay (r = 0.327, p = 0.034) and Nslain (r = 0.418, p = 0.008). Collinearity among the included parameters was assessed using the variation inflation factor (VIF). In cases where more than one significant predictor was identified, mediation and moderation analyses were used to examine the relationships between/among the significant predictors, controlling for the effects of all the other variables included in the MLR model.Normality of all data was verified with the Shapiro–Wilk tests. A p ≤ 0.05, corrected if applicable, was considered to be statistically significant. All statistical analyses were performed with the software package SPSS 20.0 (PC version).
3. Results
A total of 42 subjects were included in this study. Table 1 lists the demographic and in-game behavioral parameters of these subjects.
3.1. Analysis 1: Game Phase-Dependent Changes of In-Game HRV
The High-Risk group and Low-Risk group showed significant inter-group difference in CIAS-R (t = −10.865, p < 0.001), but not in any other demographic parameters (Table 1). Neither did The two groups show any statistically significant inter-group differences in game length, Nslay or Nslain (Table 1).Analyzing the data with RM-ANCOVA yielded statistically significant main effect of group for LF/HF (F(1,37) = 4.069, p = 0.050, η2 = 0.099, Figure 1a), but not for LF (F(1,37) = 0.303, p = 0.586, η2 = 0.008, Figure 1b), HF (F(1,37) = 2.519, p = 0.121, η2 = 0.064, Figure 1c) or any other HRV indices. A statistically significant main effect of game phase × group interaction was observed for STRESS (F(1,37) = 3.285, p = 0.043, η2 = 0.082). STRESS tended to be higher in EG than in MG (p = 0.073, FDR corrected) in the High-Risk group, while the Low-Risk Group did not show this trend (Figure 1d). No HRV index showed statistically significant main effects of game phase or game phase × covariant interactions, suggesting that statistically in-game HRV was not game phase-dependent.
3.2. Analysis 2: Multiple Linear Regression of HRV Measured over WG
The results of enter MLR analysis of HRV measured over WG are listed in Table 2. The prediction models for HF (R2 = 0.392, F(6,35) = 3.761, p = 0.005), RMSSD (R2 = 0.390, F(6,35) = 3.731, p = 0.006) and STRESS (R2 = 0.303, F(6,35) = 2.534, p = 0.038) were statistically significant. HF was predicted by CIAS-R (β = −0.279, p = 0.040), ranking score (β = −0.472, p = 0.009) and Nslain (β = −0.532, p = 0.003). RMSSD was predicted by CIAS-R (β = −0.265, p = 0.047) and Nslain (β = −0.560, p = 0.002). STRESS was predicted by, and only by, Nslain (β = 0.519, p = 0.006). Without having statistically significant prediction models, LF/HF (β = 0.430, p = 0.008) was predicted by, and only by, CIAS-R. SDNN (β = −0.545, p = 0.005), LF (β = −0.517, p = 0.009) and TP (β = −0.562, p = 0.004) was predicted by, and only by, Nslain. All parameters included in the regression analysis had a VIF < 5, indicating that there was no significant collinearity among them in the regression analyses 62. Figure 2 plots LF/HF, LF, HF and STRESS against CIAS-R and Nslain, as well as the linear fits to the data.Neither the HF vs. CIAS-R correlation nor the RMSSD vs. CIAS-R correlation was moderated/mediated by NSlain, nor was the HF vs. CIAS-R correlation moderated/mediated by ranking score. However, the correlation between HF and ranking score was found to be partially mediated by Nslain, and vice versa. The mediating effects of Nslain (total effect = −1.950, p = 0.407; direct effect = −6.908, p = 0.010; indirect effect = 4.958, p < 0.001) and ranking score (total effect = −2.173, p = 0.056; direct effect = −4.175, p = 0.002; indirect effect = 2.002, p < 0.001) on each other manifested as a masking effect, given the opposite signs of the direct and indirect effects 63. Assessing pairwise correlations among the parameters entered into MLR yielded a negative correlation between Nslain and ranking score (r = −0.507, p = 0.001, Spearman’s correlation), which was also the only pairwise correlation that was statistically significant.
4. Discussion
The current study aimed to characterize in-game HRV in male university student HGs when they played a round of an LOL game, and explore how the in-game HRV was modulated by human characteristics, especially CIAS-R, and in-game performance of the player. When dividing the players into the High-Risk and Low-Risk groups based on CIAS-R, it was shown that the High-Risk group had significantly higher LF/HF during gameplay, regardless of the game phase. The players in the High-Risk group, but not in the Low-Risk group, also tended to have higher STRESS in EG than in MG. Analyzing the data from all players together, a significant predictor for in-game HRV indices measured over WG was identified with enter MLR. LF/HF was found to be predicted by, and only by, CIAS-R. In comparison, HRV indices commonly used as indicators of sympathetic activity, such as STRESS, SDNN and TP, were predicted by, and only by, NSlain. HF and RMSSD, two HRV indices commonly used as indicators of parasympathetic activity, were predicted by both CIAS-R and Nslain, and additionally HF was also predicted by ranking score. HoWP had little influence on in-game HRV.The results of the two analyses demonstrated, convergently, that the players at high risk of developing IGD had higher LF/HF during gameplay (Figure 1a and Table 2). HF/LF is an HRV index frequently used to assess the balance between the sympathetic and parasympathetic branches of the ANS 59,64,65. Previous research has reported that individuals with IGD have higher LF/HF at rest compared to healthy controls 37,47. Interestingly, individuals with alcohol and methamphetamine dependence are also known to have higher LF/HF at rest, presumably reflecting a shifted autonomic balance towards a sympathetically dominated state 66 or cardiotoxic/neurotoxic autonomic dysbalance 67. The results obtained in this study are consistent with and expand upon these previous findings, by showing that the players at high risk of developing IGD (i.e., CIAS-R > 63), but yet to be officially diagnosed with IGD, had elevated LF/HF during online video game playing. Moreover, it was demonstrated that LF/HF was higher in the players at high risk of developing IGD in all three game phases, suggesting that this feature may not be less related to the task load and cognitive demand of gameplay, but rather a personal attribute of the player, which would manifest both at rest and during gameplay.Elevated in-game LF/HF in the players at high risk of developing IGD appeared to be driven mainly by reduced HF. In Analysis 1, HF appeared to be lower in the High-Risk group than in the Low-Risk group (Figure 1c), although the difference did not reach statistical significance. In Analysis 2, CIAS-R was identified to be a significant predictor of HF, but not of LF (Table 2). Like RMSSD, HF is a well-established indicator of parasympathetic (vagal) tone 64,68. In comparison, the interpretation of LF is more complicated. It is argued by some authors that LF is related to both sympathetic and parasympathetic activities 65,69. Both HF and RMSSD were negatively predicted by CIAS-R, suggesting that in-game parasympathetic activity was lower in the players at high risk of developing IGD. This observation is consistent with previous reports showing that only subjects with IGD, but not healthy controls, had significant HF decreases during gameplay compared to pre-game baseline 48,49.STRESS is sensitive to amplification of sympathetic tone and considered an HRV index reflecting the activity of sympathetic regulation gears 59. In Analysis 1, the High-Risk group showed higher STRESS in EG than in MG (Figure 1d). This observation is in line with previous reports showing that individuals with IGD have significant decreases in HF during the first 5 min of LOL playing 48,53. On the other hand, it seemingly contradicts the finding of Hong et al., who reported no significant HF changes in the first 5 min in the IGD subjects 49. The mechanisms underlying game phase-related sympathetic/parasympathetic activation in the players at high risk of developing IGD remain to be elucidated. Increased reactivity to game cues, such as anticipation and anxiety, might have given rise to elevated STRESS in EG in the players at high risk of developing IGD. Previous studies have demonstrated that exposure to visual game cues is sufficient to induce significant sympathetic activations in individuals with IGD, manifesting as increased respiratory rate and decreased standard deviation of HR 70. Upon exposure to game cues, individuals with IGD also exhibited higher activations in distributed brain regions in the fronto–striatal–limbic networks, which are known to be involved in planning, motivational encoding and reward processing 71,72,73. Attenuated impulse control might have contributed to enhanced reactivity of the CNS and ANS to game cues in these individuals 74,75,76.A number of HRV indices were found to be predicted significantly by Nslain, including both the indicators of parasympathetic (i.e., HF and RMSSD) activity and those of sympathetic (i.e., STRESS, SDNN and TP) activity. STRESS increases with sympathetic activation 59, and TP and SDNN decrease with sympathetic activation 77,78,79. Collectively, the results listed in Table 2 suggest that in-game sympathetic activity increases with NSlain, while in-game parasympathetic activity decrease with NSlain. Slain is generally considered as a negative event in LOL. The player showing a count of slain that is above the team average is often considered an easy pick and often targeted at by the opponent team deliberately. At the same time, he or she may face destructive criticism from the teammates and a risk of being reported as a feeder or griefer. Nslain represents the total number of times a player is slain in WG. Therefore, having a high Nslain, for most of the time, would mean the player has had poor performance and unpleasant experience in the game.Numerous studies have shown that task-related changes in HRV are modulated by physical/cognitive demands, subjectively perceived difficulty, mental stress and task performance 31,80,81,82. Consistently, these studies show that tasks with higher difficulty and heavier cognitive demand are in general associated with higher level of mental stress, augmented sympathetic activations and weakened parasympathetic activities 31,83,84. Our observations concerning the prediction of in-game HRV by NSlain are consistent with these previous findings, supporting the notion that the poorer the player’s performance during gameplay, the more stressed he/she may feel, consequently leading to enhanced sympathetic activities and reduced parasympathetic activities. While the in-game sympathetic activity was mainly determined how well the player performed during gameplay, the in-game parasympathetic (vagal) activity is modulated by both behavioral performance and personal attributes of the player, as evidenced by the prediction of RMSSD and HF by both NSlain and CIAS-R. These results suggest that the sympathetic and parasympathetic branches are likely regulated through dissociable psychophysiological pathways during online video game playing, with the parasympathetic branch more closely related to the propensity of developing IGD.Additionally, HF was negatively predicted by ranking score (Table 2). It should be noted that NSlain correlated negatively with ranking score as well, and the correlation between HF and ranking score was found to be partially mediated by NSlain, and vice versa. Both the direct effects and indirect effects of ranking score and NSlain on HF were statistically significant, albeit in opposite signs. This means that ranking score and NSlain can modulate HF significantly and independently, despite that the effects of the two tend to mask each other. In other words, the high-ranking players would show an in-game HF lower than that observed in the low-ranking players, when they have the same NSlain; and the players with a high NSlain would have an in-game HF lower than that observed in those with a low NSlain, when they have the same ranking score.The negative correlation between ranking score and in-game HF could have arisen from the game mechanics of LOL. The matchmaking algorithm of LOL summons only those with comparable gaming records and skill levels to play together in a game, and in principle this practice customizes the game difficulty for each participant. However, the gameplay intensity and cognitive load in absolute terms may, still, vary among the games played by the players at different ranks. In an MOBA game, the collective actions of all players influence how the game unfolds and how competitive it is. It therefore may be true that the high-ranking players need to be more immersed and use higher and more complicated cognitive exertions, such as extra vigilance, divided attention and multi-tasking, to achieve a favorable outcome, as the margins for lapses and errors are narrower for them than for those at lower ranks. It is known that increases in immersion (i.e., flow), cognitive demand and “adrenaline-rush” experience during video game playing give rise to the so-called “flow” state, which is characterized by parasympathetic withdrawal-associated arousal and a decrease in HF 85,86,87,88. Consistent with our observation, previous studies have demonstrated that expert neurointerventionalists have lower HF than non-experts when performing simulated neuroangiographies with the same workload 89, and familiarized chess players have lower HF than unfamiliarized chess players when playing chess in a strange computer environment 30. It should be noted that there are a number of reports in the literature showing that experts tend to have higher HF than non-experts at rest 90,91 and the individuals having a higher HF at rest often display superior performance in cognitive tasks, such as N-back task and Stroop task 92,93. High-performance chess players are shown to have higher RMSSD than low-performance chess players when playing chess at low- to medium-difficulty levels, but not at high-difficulty levels 31. These results highlight that task-related changes in parasympathetic activity are most likely modulated by the complex interactions among the expertise, task difficulty and contingency of behavioral performance, rather than expertise per se.There are several limitations that need to be addressed. Firstly, complementary measurements of physiological indices such as respiration rate, blood pressure and skin conductance are required to provide a more complete and comprehensive understanding on how in-game autonomic activities are affected by in-game behaviors and personal attributes of the player. For example, respiratory parameters are important determinants of respiratory sinus arrhythmia (RSA), which may affect LF and HF independent of changes in cardiac vagal tone. Decreased respiratory rate is usually accompanied with increased LF 94. Therefore, the possible contribution of altered respiratory rhythm to the relatively higher LF/HF observed in the players at high risk of developing IGD cannot be excluded, given the reports showing that these players tended to have a lower respiration rate and/or hold breath more often during VGP 95. Further studies are needed to clarify how changes of breath rhythm affect the measurement and interpretation of in-game HRV. Secondly, since baseline HRV was not measured in this study, it remained unclear to what extent in-game HRV was related to and influenced by resting state HRV. A, LOL game typically lasts 20–40 min. Our preliminary experiments showed that it is challenging for subjects to remain in a resting state for an extended period of time that is comparable to the actual gameplay (i.e., tens of minutes). Thirdly, the data were obtained from a relatively small and homogenous group of players who played a single game. Further research is required to investigate whether the observed results can be generalized to other player populations and games. Finally, the precision, accuracy and interpretation of HRV measurements are known to depend on the length of the time window during which the heart beats are recorded 79. HRV measurements at various timescales may capture different aspects of in-game autonomic changes and their associations with in-game behaviors and psychophysiological states 96.
5. Conclusions
HRV measurements were used to detect changes in autonomic activities during online video game playing in male university student HGs. In-game LF/HF, an HRV indictor of autonomic balance, was found to be higher in the players at high risk of developing IGD, giving further support to the notion that this HRV index may potentially be used for IGD screening and/or diagnosis. Poor performance during gameplay was found to be linked to higher sympathetic activity and lower parasympathetic activity. The in-game performance also, in part, mediated the modulating effect of ranking score on HRV indicators of parasympathetic activity, including HF. Last but not least, the players at high risk of developing IGD tended to have higher STRESS in EG than MG, probably reflecting enhanced reactivity to game cues in this population. Together, the results of this study suggest that IGD-related autonomic dysregulation may have arisen from the complex interactions among personal attributes and in-game behavioral performance of the player, as well as factors embedded in the mechanics of the game being played.
Author Contributions
Conceptualization, K.L., X.Z. and H.L.; methodology, K.L. and N.W.; investigation, K.L., X.Z. and N.W.; writing—original draft preparation, K.L.; writing—review and editing, H.L.; visualization, K.L.; supervision, H.L.; funding acquisition, H.L. All authors have read and agreed to the published version of the manuscript.
Funding
This work was supported by a grant from the National Natural Science Foundation of China (21790392).
Institutional Review Board Statement
This study protocol was approved by the Human Subjects Institutional Review Board of Wuhan Institute of Physics and Mathematics, Chinese Academy of Science (WIPM20190303) (approved date 3 March 2019). All patients receive written information about the research project, benefits and risks of participation. They are informed that they can withdraw from the study at any time. Written informed consent was obtained prior to intake assessment.
Informed Consent Statement
Informed consent was obtained from all participants involved in the study.
Data Availability Statement
The data presented in this study are available on request from the corresponding author. The data are not publicly available due to the fact that they constitute an excerpt of research in progress.
Conflicts of Interest
The authors declare no conflict of interest.
References
- Reed, G.M.; First, M.B.; Kogan, C.S.; Hyman, S.E.; Gureje, O.; Gaebel, W.; Maj, M.; Stein, D.J.; Maercker, A.; Tyrer, P.; et al. Innovations and changes in the ICD-11 classification of mental, behavioural and neurodevelopmental disorders. World Psychiatry2019, 18, 3–19. Google Scholar CrossRef PubMed
- Ko, C.H.; Hsieh, T.J.; Wang, P.W.; Lin, W.C.; Yen, C.F.; Chen, C.S.; Yen, J.Y. Altered gray matter density and disrupted functional connectivity of the amygdala in adults with Internet gaming disorder. Prog. Neuro-Psychopharmacol. Biol. Psychiatry2015, 57, 185–192. Google Scholar CrossRef PubMed
- Lin, X.; Dong, G.; Wang, Q.; Du, X. Abnormal gray matter and white matter volume in ‘internet gaming addicts’. Addict. Behav.2015, 40, 137–143. Google Scholar CrossRef PubMed
- Jin, C.; Zhang, T.; Cai, C.; Bi, Y.; Li, Y.; Yu, D.; Zhang, M.; Yuan, K. Abnormal prefrontal cortex resting state functional connectivity and severity of internet gaming disorder. Brain Imaging Behav.2016, 10, 719–729. Google Scholar CrossRef PubMed
- Yuan, K.; Qin, W.; Yu, D.; Bi, Y.; Xing, L.; Jin, C.; Tian, J. Core brain networks interactions and cognitive control in internet gaming disorder individuals in late adolescence/early adulthood. Brain Struct. Funct.2016, 221, 1427–1442. Google Scholar CrossRef PubMed
- Zhang, J.T.; Yao, Y.W.; Li, C.S.; Zang, Y.F.; Shen, Z.J.; Liu, L.; Wang, L.J.; Liu, B.; Fang, X.Y. Altered resting-state functional connectivity of the insula in young adults with internet gaming disorder. Addict. Biol.2016, 21, 743–751. Google Scholar CrossRef PubMed
- Chen, C.Y.; Yen, J.Y.; Wang, P.W.; Liu, G.C.; Yen, C.F.; Ko, C.H. Altered Functional Connectivity of the Insula and Nucleus Accumbens in Internet Gaming Disorder: A Resting State fMRI Study. Eur. Addict. Res.2016, 22, 192–200. Google Scholar CrossRef PubMed
- Dong, G.; Shen, Y.; Huang, J.; Du, X. Impaired error-monitoring function in people with internet addiction disorder: An event-related fMRI study. Eur. Addict. Res.2013, 19, 269–275. Google Scholar CrossRef
- Shin, Y.B.; Kim, H.; Kim, S.J.; Kim, J.J. A neural mechanism of the relationship between impulsivity and emotion dysregulation in patients with Internet gaming disorder. Addict. Biol.2021, 26, e12916. Google Scholar CrossRef
- Wang, Y.; Hu, Y.; Xu, J.; Zhou, H.; Lin, X.; Du, X.; Dong, G. Dysfunctional prefrontal function is associated with impulsivity in people with internet gaming disorder during a delay discounting task. Front. Psychiatry2017, 8, 287. Google Scholar CrossRef
- Cheng, Y.C.; Huang, Y.C.; Huang, W.L. Can heart rate variability be viewed as a biomarker of problematic internet use? a systematic review and meta-analysis. Appl. Psychophysiol. Biofeedback2023, 48, 1–10. Google Scholar CrossRef PubMed
- Lu, D.W.; Wang, J.W.; Huang, A.C. Differentiation of internet addiction risk level based on autonomic nervous responses: The internet-addiction hypothesis of autonomic activity. Cyberpsychol. Behav. Soc. Netw.2010, 13, 371–378. Google Scholar CrossRef PubMed
- Spada, M.M. An overview of problematic internet use. Addict. Behav.2014, 39, 3–6. Google Scholar CrossRef PubMed
- de Zambotti, M.; Trinder, J.; Silvani, A.; Colrain, I.M.; Baker, F.C. Dynamic coupling between the central and autonomic nervous systems during sleep: A review. Neurosci. Biobehav. Rev.2018, 90, 84–103. Google Scholar CrossRef
- Patron, E.; Mennella, R.; Messerotti Benvenuti, S.; Thayer, J.F. The frontal cortex is a heart-brake: Reduction in delta oscillations is associated with heart rate deceleration. NeuroImage2019, 188, 403–410. Google Scholar CrossRef PubMed
- Thayer, J.F.; Hansen, A.L.; Saus-Rose, E.; Johnsen, B.H. Heart rate variability, prefrontal neural function, and cognitive performance: The neurovisceral integration perspective on self-regulation, adaptation, and health. Ann. Behav. Med.2009, 37, 141–153. Google Scholar CrossRef
- Shaffer, F.; McCraty, R.; Zerr, C.L. A healthy heart is not a metronome: An integrative review of the heart’s anatomy and heart rate variability. Front. Psychol.2014, 5, 1040. Google Scholar CrossRef
- Al, E.; Iliopoulos, F.; Forschack, N.; Nierhaus, T.; Grund, M.; Motyka, P.; Gaebler, M.; Nikulin, V.V.; Villringer, A. Heart-brain interactions shape somatosensory perception and evoked potentials. Proc. Natl. Acad. Sci. USA2020, 117, 10575–10584. Google Scholar CrossRef
- Barber, A.D.; John, M.; DeRosse, P.; Birnbaum, M.L.; Lencz, T.; Malhotra, A.K. Parasympathetic arousal-related cortical activity is associated with attention during cognitive task performance. NeuroImage2020, 208, 116469. Google Scholar CrossRef
- Candia-Rivera, D.; Catrambone, V.; Thayer, J.F.; Gentili, C.; Valenza, G. Cardiac sympathetic-vagal activity initiates a functional brain-body response to emotional arousal. Proc. Natl. Acad. Sci. USA2022, 119, e2119599119. Google Scholar CrossRef
- Whitehurst, L.N.; Cellini, N.; McDevitt, E.A.; Duggan, K.A.; Mednick, S.C. Autonomic activity during sleep predicts memory consolidation in humans. Proc. Natl. Acad. Sci. USA2016, 113, 7272–7277. Google Scholar CrossRef PubMed
- Underwood, E. A sense of self-communication between the brain and other organs shapes how we think, remember, and feel. Science2021, 372, 1142–1145. Google Scholar CrossRef PubMed
- Thayer, J.F.; Ahs, F.; Fredrikson, M.; Sollers, J.J., 3rd; Wager, T.D. A meta-analysis of heart rate variability and neuroimaging studies: Implications for heart rate variability as a marker of stress and health. Neurosci. Biobehav. Rev.2012, 36, 747–756. Google Scholar CrossRef PubMed
- Camm, A.J.; Malik, M.; Bigger, J.T.; Breithardt, G.; Cerutti, S.; Cohen, R.J.; Coumel, P.; Fallen, E.L.; Kennedy, H.L.; Kleiger, R. Heart rate variability. Standards of measurement, physiological interpretation, and clinical use. Eur. Heart J.1996, 17, 354–381. Google Scholar CrossRef
- Zhang, X.; Long, K.; Wang, N.; Zhang, J.; Lei, H. Synchronous measurements of prefrontal activity and pulse rate variability during online video game playing with functional near-infrared spectroscopy. J. Innov. Opt. Health Sci.2023, 16, 1–15. Google Scholar CrossRef
- Sammito, S.; Thielmann, B.; Seibt, R.; Klussmann, A.; Weippert, M.; Böckelmann, I. Guideline for the application of heart rate and heart rate variability in occupational medicine and occupational science. ASU Int.2015, 2015, 1–29. Google Scholar CrossRef
- Byun, S.; Kim, A.Y.; Jang, E.H.; Kim, S.; Choi, K.W.; Yu, H.Y.; Jeon, H.J. Detection of major depressive disorder from linear and nonlinear heart rate variability features during mental task protocol. Comput. Biol. Med.2019, 112, 103381. Google Scholar CrossRef
- Keary, T.A.; Hughes, J.W.; Palmieri, P.A. Women with posttraumatic stress disorder have larger decreases in heart rate variability during stress tasks. Int. J. Psychophysiol.2009, 73, 257–264. Google Scholar CrossRef
- Yeo, M.; Lim, S.; Yoon, G. Analysis of biosignals during immersion in computer games. J. Med. Syst.2017, 42, 3. Google Scholar CrossRef
- Fuentes-Garcia, J.P.; Pereira, T.; Castro, M.A.; Carvalho Santos, A.; Villafaina, S. Heart and brain responses to real versus simulated chess games in trained chess players: A quantitative EEG and HRV study. Int. J. Environ. Res. Public Health2019, 16, 5021. Google Scholar CrossRef
- Fuentes-Garcia, J.P.; Villafaina, S.; Collado-Mateo, D.; de la Vega, R.; Olivares, P.R.; Clemente-Suarez, V.J. Differences between high vs. low performance chess players in heart rate variability during chess problems. Front. Psychol.2019, 10, 409. Google Scholar CrossRef PubMed
- Thielmann, B.; Hartung, J.; Bockelmann, I. Objective assessment of mental stress in individuals with different levels of effort reward imbalance or overcommitment using heart rate variability: A systematic review. Syst. Rev.2022, 11, 48. Google Scholar CrossRef PubMed
- Marin-Morales, J.; Higuera-Trujillo, J.L.; Guixeres, J.; Llinares, C.; Alcaniz, M.; Valenza, G. Heart rate variability analysis for the assessment of immersive emotional arousal using virtual reality: Comparing real and virtual scenarios. PLoS ONE2021, 16, e0254098. Google Scholar CrossRef PubMed
- Goncalves, S.F.; Chaplin, T.M.; Lopez, R., Jr.; Regalario, I.M.; Niehaus, C.E.; McKnight, P.E.; Stults-Kolehmainen, M.; Sinha, R.; Ansell, E.B. High-frequency heart rate variability and emotion-driven impulse control difficulties during adolescence: Examining experienced and expressed negative emotion as moderators. J. Early Adolesc.2021, 41, 1151–1176. Google Scholar CrossRef PubMed
- Sakuragi, S.; Sugiyama, Y. Effects of reward and punishment on task performance, mood and autonomic nervous function, and the interaction with personality. J. Physiol. Anthropol.2009, 28, 181–190. Google Scholar CrossRef PubMed
- Moon, E.; Lee, S.H.; Kim, D.H.; Hwang, B. Comparative study of heart rate variability in patients with schizophrenia, bipolar disorder, post-traumatic stress disorder, or major depressive disorder. Clin. Psychopharmacol. Neurosci.2013, 11, 137–143. Google Scholar CrossRef PubMed
- Lin, P.C.; Kuo, S.Y.; Lee, P.H.; Sheen, T.C.; Chen, S.R. Effects of internet addiction on heart rate variability in school-aged children. J. Cardiovasc. Nurs.2014, 29, 493–498. Google Scholar CrossRef PubMed
- Kim, N.; Hughes, T.L.; Park, C.G.; Quinn, L.; Kong, I.D. Altered autonomic functions and distressed personality traits in male adolescents with internet gaming addiction. Cyberpsychol. Behav. Soc. Netw.2016, 19, 667–673. Google Scholar CrossRef
- Moretta, T.; Buodo, G. Autonomic stress reactivity and craving in individuals with problematic internet use. PLoS ONE2018, 13, e0190951. Google Scholar CrossRef
- Moretta, T.; Sarlo, M.; Buodo, G. Problematic internet use: The relationship between resting heart rate variability and emotional modulation of inhibitory control. Cyberpsychol. Behav. Soc. Netw.2019, 22, 500–507. Google Scholar CrossRef
- Park, S.M.; Lee, J.Y.; Choi, A.R.; Kim, B.M.; Chung, S.J.; Park, M.; Kim, I.Y.; Park, J.; Choi, J.; Hong, S.J.; et al. Maladaptive neurovisceral interactions in patients with internet gaming disorder: A study of heart rate variability and functional neural connectivity using the graph theory approach. Addict. Biol.2020, 25, e12805. Google Scholar CrossRef PubMed
- Friedman, B.H. An autonomic flexibility-neurovisceral integration model of anxiety and cardiac vagal tone. Biol. Psychol.2007, 74, 185–199. Google Scholar CrossRef PubMed
- Kuiper, M.W.M.; Verhoeven, E.W.M.; Geurts, H.M. Heart rate variability predicts inhibitory control in adults with autism spectrum disorders. Biol. Psychol.2017, 128, 141–152. Google Scholar CrossRef PubMed
- Hwang, S.; Martins, J.S.; Douglas, R.J.; Choi, J.J.; Sinha, R.; Seo, D. Irregular autonomic modulation predicts risky drinking and altered ventromedial prefrontal cortex response to stress in alcohol use disorder. Alcohol. Alcohol.2021, 57, 437–444. Google Scholar CrossRef PubMed
- Blanchard, E.B.; Wulfert, E.; Freidenberg, B.M.; Malta, L.S. Psychophysiological assessment of compulsive gamblers’ arousal to gambling cues: A pilot study. Appl. Psychophysiol. Biofeedback2000, 25, 155–165. Google Scholar CrossRef PubMed
- Hsieh, D.L.; Hsiao, T.C. Heart rate variability of internet gaming disorder addicts in emotional states. In Proceedings of the 2016 International Conference on Biomedical Engineering (BME-HUST), Hanoi, Vietnam, 5–6 October 2016; pp. 155–158. Google Scholar
- Chang, J.S.; Kim, E.Y.; Jung, D.; Jeong, S.H.; Kim, Y.; Roh, M.S.; Ahn, Y.M.; Hahm, B.J. Altered cardiorespiratory coupling in young male adults with excessive online gaming. Biol. Psychol.2015, 110, 159–166. Google Scholar CrossRef PubMed
- Lee, D.; Park, J.; Namkoong, K.; Hong, S.J.; Kim, I.Y.; Jung, Y.C. Diminished cognitive control in internet gaming disorder: A multimodal approach with magnetic resonance imaging and real-time heart rate variability. Prog. Neuro-Psychopharmacol. Biol. Psychiatry2021, 111, 110127. Google Scholar CrossRef
- Hong, S.J.; Lee, D.; Park, J.; Namkoong, K.; Lee, J.; Jang, D.P.; Lee, J.E.; Jung, Y.C.; Kim, I.Y. Altered heart rate variability during gameplay in internet gaming disorder: The impact of situations during the game. Front. Psychiatry2018, 9, 429. Google Scholar CrossRef
- Lin, T.C. Effects of gender and game type on autonomic nervous system physiological parameters in long-hour online game players. Cyberpsychol. Behav. Soc. Netw.2013, 16, 820–827. Google Scholar CrossRef
- Ivarsson, M.; Anderson, M.; Akerstedt, T.; Lindblad, F. Playing a violent television game affects heart rate variability. Acta Paediatr.2009, 98, 166–172. Google Scholar CrossRef
- Porter, A.M.; Goolkasian, P. Video games and stress: How stress appraisals and game content affect cardiovascular and emotion outcomes. Front. Psychol.2019, 10, 967. Google Scholar CrossRef PubMed
- Lee, D.; Hong, S.J.; Jung, Y.C.; Park, J.; Kim, I.Y.; Namkoong, K. Altered heart rate variability during gaming in internet gaming disorder. Cyberpsychol. Behav. Soc. Netw.2018, 21, 259–267. Google Scholar CrossRef PubMed
- Neubauer, A.C.; Fink, A. Intelligence and neural efficiency. Neurosci. Biobehav. Rev.2009, 33, 1004–1023. Google Scholar CrossRef PubMed
- Filho, E.; Husselman, T.A.; Zugic, L.; Penna, E.; Taneva, N. Performance gains in an open skill video-game task: The role of neural efficiency and neural proficiency. Appl. Psychophysiol. Biofeedback2022, 47, 239–251. Google Scholar CrossRef PubMed
- Ko, C.H.; Yen, J.Y.; Yen, C.F.; Chen, C.C.; Yen, C.N.; Chen, S.H. Screening for internet addiction: An empirical study on cut-off points for the chen internet addiction scale. Kaohsiung J. Med. Sci.2005, 21, 545–551. Google Scholar CrossRef PubMed
- Gilgen-Ammann, R.; Schweizer, T.; Wyss, T. RR interval signal quality of a heart rate monitor and an ECG Holter at rest and during exercise. Eur. J. Appl. Physiol.2019, 119, 1525–1532. Google Scholar CrossRef PubMed
- Tarvainen, M.P.; Niskanen, J.P.; Lipponen, J.A.; Ranta-Aho, P.O.; Karjalainen, P.A. Kubios HRV–heart rate variability analysis software. Comput. Methods Programs Biomed.2014, 113, 210–220. Google Scholar CrossRef
- Baevsky, R.; Berseneva, A. Use KARDiVAR system for determination of the stress level and estimation of the body adaptability: Standards of measurements and physiological interpretation. Kardivar. TV2009, 1149, d557. Available online: https://pdfs.semanticscholar.org/74a2/92bfafca4fdf1149d557348800fcc1b0f33b.pdf (accessed on 25 December 2023).
- Maher, B. Can a video game company tame toxic behaviour. Nature2016, 531, 568–571. Google Scholar CrossRef
- Rehbein, F.; King, D.L.; Staudt, A.; Hayer, T.; Rumpf, H.-J. Contribution of game genre and structural game characteristics to the risk of problem gaming and gaming disorder: A aystematic review. Curr. Addic. Rep.2021, 8, 263–281. Google Scholar CrossRef
- Akinwande, M.O.; Dikko, H.G.; Samson, A. Variance inflation factor: As a condition for the inclusion of suppressor variable(s) in regression analysis. Open J. Stat.2015, 05, 754–767. Google Scholar CrossRef
- MacKinnon, D.P.; Krull, J.L.; Lockwood, C.M. Equivalence of the mediation, confounding and suppression effect. Prev. Sci.2000, 1, 173–181. Google Scholar CrossRef PubMed
- Malliani, A.; Pagani, M.; Lombardi, F.; Cerutti, S. Cardiovascular neural regulation explored in the frequency domain. Circulation1991, 84, 482–492. Google Scholar CrossRef PubMed
- Reyes del Paso, G.A.; Langewitz, W.; Mulder, L.J.; van Roon, A.; Duschek, S. The utility of low frequency heart rate variability as an index of sympathetic cardiac tone: A review with emphasis on a reanalysis of previous studies. Psychophysiology2013, 50, 477–487. Google Scholar CrossRef PubMed
- Jochum, T.; Reinhard, M.; Boettger, M.K.; Piater, M.; Bar, K.J. Impaired cerebral autoregulation during acute alcohol withdrawal. Drug Alcohol Depend.2010, 110, 240–246. Google Scholar CrossRef PubMed
- Henry, B.L.; Minassian, A.; Perry, W. Effect of methamphetamine dependence on heart rate variability. Addict. Biol.2012, 17, 648–658. Google Scholar CrossRef PubMed
- Mietus, J.E.; Peng, C.K.; Henry, I.; Goldsmith, R.L.; Goldberger, A.L. The pNNx files: Re-examining a widely used heart rate variability measure. Heart2002, 88, 378–380. Google Scholar CrossRef PubMed
- Watson, A.M.; Hood, S.G.; Ramchandra, R.; McAllen, R.M.; May, C.N. Increased cardiac sympathetic nerve activity in heart failure is not due to desensitization of the arterial baroreflex. Am. J. Physiol.-Heart C2007, 293, H798–H804. Google Scholar CrossRef
- Kim, H.; Ha, J.; Chang, W.D.; Park, W.; Kim, L.; Im, C.H. Detection of craving for gaming in adolescents with internet gaming disorder using multimodal biosignals. Sensors2018, 18, 102. Google Scholar CrossRef
- Liu, L.; Yip, S.W.; Zhang, J.T.; Wang, L.J.; Shen, Z.J.; Liu, B.; Ma, S.S.; Yao, Y.W.; Fang, X.Y. Activation of the ventral and dorsal striatum during cue reactivity in internet gaming disorder. Addict. Biol.2017, 22, 791–801. Google Scholar CrossRef
- Zhang, J.T.; Yao, Y.W.; Potenza, M.N.; Xia, C.C.; Lan, J.; Liu, L.; Wang, L.J.; Liu, B.; Ma, S.S.; Fang, X.Y. Effects of craving behavioral intervention on neural substrates of cue-induced craving in internet gaming disorder. NeuroImage Clin.2016, 12, 591–599. Google Scholar CrossRef PubMed
- Zhang, J.; Chen, S.; Jiang, Q.; Dong, H.; Zhao, Z.; Du, X.; Dong, G.-H. Disturbed craving regulation to gaming cues in internet gaming disorder: Implications for uncontrolled gaming behaviors. J. Psychiatr. Res.2021, 140, 250–259. Google Scholar CrossRef PubMed
- Wang, Y.; Wu, L.; Wang, L.; Zhang, Y.; Du, X.; Dong, G. Impaired decision-making and impulse control in Internet gaming addicts: Evidence from the comparison with recreational internet game users. Addict. Biol.2017, 22, 1610–1621. Google Scholar CrossRef PubMed
- Zha, R.; Tao, R.; Kong, Q.; Li, H.; Liu, Y.; Huang, R.; Wei, Z.; Hong, W.; Wang, Y.; Zhang, D.; et al. Impulse control differentiates internet gaming disorder from non-disordered but heavy internet gaming use: Evidence from multiple behavioral and multimodal neuroimaging data. Comput. Hum. Behav.2022, 130, 107184. Google Scholar CrossRef
- Brand, M.; Rumpf, H.-J.; Demetrovics, Z.; King, D.L.; Potenza, M.N.; Wegmann, E. Gaming disorder is a disorder due to addictive behaviors: Evidence from behavioral and neuroscientific studies addressing cue reactivity and craving, executive functions, and decision-making. Curr. Addic. Rep.2019, 6, 296–302. Google Scholar CrossRef
- Montano, N.; Porta, A.; Cogliati, C.; Costantino, G.; Tobaldini, E.; Casali, K.R.; Iellamo, F. Heart rate variability explored in the frequency domain: A tool to investigate the link between heart and behavior. Neurosci. Biobehav. Rev.2009, 33, 71–80. Google Scholar CrossRef PubMed
- Stein, P.K.; Bosner, M.S.; Kleiger, R.E.; Conger, B.M. Heart rate variability: A measure of cardiac autonomic tone. Am. Heart J.1994, 127, 1376–1381. Google Scholar CrossRef PubMed
- Shaffer, F.; Ginsberg, J.P. An overview of heart rate variability metrics and norms. Front. Public Health2017, 5, 258. Google Scholar CrossRef
- Kim, H.G.; Cheon, E.J.; Bai, D.S.; Lee, Y.H.; Koo, B.H. Stress and heart rate variability: A meta-analysis and review of the literature. Psychiatry Investig.2018, 15, 235–245. Google Scholar CrossRef
- Pulopulos, M.M.; Vanderhasselt, M.A.; De Raedt, R. Association between changes in heart rate variability during the anticipation of a stressful situation and the stress-induced cortisol response. Psychoneuroendocrinology2018, 94, 63–71. Google Scholar CrossRef
- Luft, C.D.; Takase, E.; Darby, D. Heart rate variability and cognitive function: Effects of physical effort. Biol. Psychol.2009, 82, 164–168. Google Scholar CrossRef PubMed
- Luque-Casado, A.; Perales, J.C.; Cárdenas, D.; Sanabria, D. Heart rate variability and cognitive processing: The autonomic response to task demands. Biol. Psychol.2016, 113, 83–90. Google Scholar CrossRef PubMed
- Taelman, J.; Vandeput, S.; Gligorijević, I.; Spaepen, A.; Van Huffel, S. Time-frequency heart rate variability characteristics of young adults during physical, mental and combined stress in laboratory environment. In Proceedings of the 2011 Annual International Conference of the IEEE Engineering in Medicine and Biology Society, Boston, MA, USA, 30 August–3 September 2011; pp. 1973–1976. Google Scholar
- Khoshnoud, S.; Alvarez Igarzabal, F.; Wittmann, M. Brain-heart interaction and the experience of flow while playing a video game. Front. Hum. Neurosci.2022, 16, 819834. Google Scholar CrossRef PubMed
- Tozman, T.; Magdas, E.S.; MacDougall, H.G.; Vollmeyer, R. Understanding the psychophysiology of flow: A driving simulator experiment to investigate the relationship between flow and heart rate variability. Comput. Hum. Behav.2015, 52, 408–418. Google Scholar CrossRef
- Kozhevnikov, M.; Li, Y.; Wong, S.; Obana, T.; Amihai, I. Do enhanced states exist? Boosting cognitive capacities through an action video-game. Cognition2018, 173, 93–105. Google Scholar CrossRef PubMed
- Kozhevnikov, M.; Strasser, A.; Abdullah, M.A. Accessing the states of enhanced cognition in a gaming context: The importance of psychophysiological arousal. Cogn. Sci.2022, 46, e13106. Google Scholar CrossRef PubMed
- Hinzmann, D.; Singer, M.; Schmelter, V.; Kreiser, K.; Gehling, K.; Strober, L.; Kirschke, J.S.; Schulz, C.M.; Schneider, F. Differences in beginner and expert neurointerventionalists” heart rate variability during simulated neuroangiographies. Interv. Neuroradiol.2022, 15910199221128439. Google Scholar CrossRef PubMed
- Mazurek, K.; Koprowska, N.; Gajewski, J.; Zmijewski, P.; Skibniewski, F.; Różanowski, K. Parachuting training improves autonomic control of the heart in novice parachute jumpers. Biocybern. Biomed. Eng.2018, 38, 181–189. Google Scholar CrossRef
- Beffara, B.; Bret, A.G.; Vermeulen, N.; Mermillod, M. Resting high frequency heart rate variability selectively predicts cooperative behavior. Physiol. Behav.2016, 164, 417–428. Google Scholar CrossRef
- Magnon, V.; Vallet, G.T.; Benson, A.; Mermillod, M.; Chausse, P.; Lacroix, A.; Bouillon-Minois, J.B.; Dutheil, F. Does heart rate variability predict better executive functioning? a systematic review and meta-analysis. Cortex2022, 155, 218–236. Google Scholar CrossRef
- Forte, G.; Favieri, F.; Casagrande, M. Heart Rate Variability and Cognitive Function: A Systematic Review. Front. Neurosci.2019, 13, 710. Google Scholar CrossRef PubMed
- Song, H.-S.; Lehrer, P.M. The effects of specific respiratory rates on heart rate and heart rate variability. Appl. Psychophysiol. Biofeedback2003, 28, 13–23. Google Scholar CrossRef PubMed
- Chiu, W.-Y.; Chen, L.-Y.; Chi, H.-M.; Hsiao, T.-C. Toward instantaneous frequency of respiration to investigate the risk of internet gaming disorder. In Proceedings of the 2021 43rd Annual International Conference of the IEEE Engineering in Medicine & Biology Society (EMBC), Virtual Conference, 1–5 November 2021; pp. 232–235. Google Scholar
- Liegeois, R.; Li, J.; Kong, R.; Orban, C.; Van De Ville, D.; Ge, T.; Sabuncu, M.R.; Yeo, B.T.T. Resting brain dynamics at different timescales capture distinct aspects of human behavior. Nat. Commun.2019, 10, 2317. Google Scholar CrossRef PubMed Figure 1. Short-term HRV measurements in early (EG), middle (MG) and late (LG) phases of the game, including LF/HF (a), LF (b), HF (c) and STRESS (d). LF/HF: low-frequency power/high-frequency power ratio, LF: low-frequency power, HF: high-frequency power, STRESS: square root of Baevsky’s stress index. p value displayed in (d) was false discovery rate corrected for multiple (n = 9) post hoc comparisons. Figure 1. Short-term HRV measurements in early (EG), middle (MG) and late (LG) phases of the game, including LF/HF (a), LF (b), HF (c) and STRESS (d). LF/HF: low-frequency power/high-frequency power ratio, LF: low-frequency power, HF: high-frequency power, STRESS: square root of Baevsky’s stress index. p value displayed in (d) was false discovery rate corrected for multiple (n = 9) post hoc comparisons.Figure 2. Plots of selected HRV indices against CIAS-R (a) and Nslain (b). LF/HF: low-frequency power/high-frequency power ratio, LF: low-frequency power, HF: high-frequency power, STRESS: square root of Baevsky’s stress index, β: standardized regression coefficient obtained from enter multiple linear regression. * p < 0.05. Solid and dashed lines: linear fits to the data, with a statistically significant β represented by a solid line, and an insignificant β represented by a dashed line. Figure 2. Plots of selected HRV indices against CIAS-R (a) and Nslain (b). LF/HF: low-frequency power/high-frequency power ratio, LF: low-frequency power, HF: high-frequency power, STRESS: square root of Baevsky’s stress index, β: standardized regression coefficient obtained from enter multiple linear regression. * p < 0.05. Solid and dashed lines: linear fits to the data, with a statistically significant β represented by a solid line, and an insignificant β represented by a dashed line.Table 1. Demographics and in-game behavioral parameters. Table 1. Demographics and in-game behavioral parameters.| Parameters | High Risk (n = 20) M (SD) | Low Risk (n = 22)M (SD) | p Value | |:-:|:-:|:-:|:-:| | Age (years) | 20.4 (2.4) | 20.0 (1.9) | 0.648 | | Education (years) | 14.8 (2.1) | 14.4 (1.7) | 0.512 | | HoWP (hours) | 13.4 (7.5) | 11.3 (6.8) | 0.348 | | Ranking score | 3.5 (1.5) | 3.7 (1.6) | 0.736 | | CIAS-R | 67.3 (3.4) | 54.6 (4.1) | <0.001 * | | Game length | 26.8 (5.7) | 27.4 (4.7) | 0.685 | | Nslay | 7.3 (4.5) | 5.3 (4.2) | 0.140 | | Nslain | 6.4 (3.6) | 5.2 (2.3) | 0.218 | M: mean, SD: standard deviation, HoWP: self-reported hours of weekly LOL playing, CIAS-R: revised Chen Internet Addiction Scale, Nslay: number of slay, Nslain: number of slain. *: p < 0.05.Table 2. Standardized regression coefficients (β) in the multiple linear regression analysis with the enter method. Table 2. Standardized regression coefficients (β) in the multiple linear regression analysis with the enter method.| Parameters | Age | CIAS-R | HoWP | Ranking Score | Nslay | Nslain | |:-:|:-:|:-:|:-:|:-:|:-:|:-:| | Mean HR | −0.184 | 0.117 | 0.094 | −0.206 | −0.180 | −0.034 | | LF/HF | 0.100 | 0.430 * | −0.034 | 0.238 | 0.070 | 0.080 | | LF | −0.129 | 0.073 | 0.012 | −0.166 | 0.048 | −0.517 * | | TP | −0.151 | 0.024 | −0.017 | −0.231 | −0.006 | −0.562 * | | SDNN | −0.160 | −0.010 | −0.021 | −0.222 | 0.012 | −0.545 * | | STRESS # | 0.121 | 0.150 | 0.078 | 0.086 | 0.004 | 0.519 * | | HF # | −0.201 | −0.279 * | 0.008 | −0.472 * | −0.114 | −0.532 * | | RMSSD # | −0.166 | −0.265 * | −0.071 | −0.252 | 0.040 | −0.560 * | | SampEn | 0.069 | 0.069 | −0.198 | −0.218 | −0.139 | −0.081 | CIAS-R: revised Chen Internet Addiction Scale, HoWP: self-reported hours of weekly LOL playing, Nslay: number of slay, Nslain: number of slain. HR: heart rate, LF/HF: low-frequency power/high-frequency power ratio, LF: low-frequency power, TP: total power, SDNN: standard deviation of inter-beat intervals, STRESS: square root of Baevsky’s stress index, HF: high-frequency power, RMSSD: root mean square differences between successive inter-beat intervals, SampEn: sample entropy. #: regression model being statistically significant, *: p < 0.05.| | Disclaimer/Publisher’s Note: The statements, opinions and data contained in all publications are solely those of the individual author(s) and contributor(s) and not of MDPI and/or the editor(s). MDPI and/or the editor(s) disclaim responsibility for any injury to people or property resulting from any ideas, methods, instructions or products referred to in the content. | |—|—| © 2023 by the authors. Licensee MDPI, Basel, Switzerland. This article is an open access article distributed under the terms and conditions of the Creative Commons Attribution (CC BY) license (https://creativecommons.org/licenses/by/4.0/). Internet gaming disorder (IGD) has been officially listed as a mental disorder in the 11th revision of the International Classification of Diseases (ICD-11) published by the World Health Organization 1. IGD is characterized by a pattern of persistent or recurrent Internet-based or offline gaming behavior that is manifested by impaired control over the behavior, giving increasing priority to gaming to the extent that it takes precedence over other life interests and daily activities; and continuing or escalating gaming despite its negative consequences 1. Numerous neuroimaging studies have demonstrated that IGD is associated with various abnormalities in the central nervous system (CNS), manifesting as structural plasticity 2,3,4 and altered resting-state functional connectivity in specific brain areas/networks 5,6,7, as well as aberrant functional activations in tasks involving executive control, impulse inhibition and working memory 8,9,10. Abnormal autonomic activities have also been reported in IGD and problematic internet use (PIU) 11,12. PIU has currently no official diagnostic criteria, but can be defined as use of Internet that created psychological, social, school and/or work difficulties in a person’s life 13. The autonomic nervous system (ANS) is coupled to the CNS anatomically and functionally 14. The ANS receives top-down regulation from the CNS 15,16, and provides feedback through the ascending pathways 17,18. The ANS plays an important role in regulating the majority of the body’s internal processes, allowing adaptive responses to internal and external stressors and guaranteeing the body’s homeostatic milieu 14. The dynamic interactions between the CNS and ANS modulate perception, cognition, emotion generation/regulation, memory consolidation and even the sense of self 16,19,20,21,22. Heart rate variability (HRV) is probably the most widely used measurement to assess ANS activity by quantifying the time interval variations between adjacent heart beats 23,24,25. Sympathetic/parasympathetic tones and prevalence are represented by different HRV indices computed in the time, frequency and non-linear domains 26. HRV measurements have been used in laboratory 27,28 and real-world tasks 29,30 to assess ANS activity perturbations associated with cognitive load 31, mental stress 32, emotional arousal 33, executive control 34 and reactivity to reward 35. Moreover, it has been proposed that HRV can serve as an auxiliary diagnostic tool for certain mental diseases 27,36. Compared to none-gamer or occasional/habitual player controls without IGD, players with IGD were reported to have higher heart rates (HRs), lower standard deviation of inter-beat intervals (SDNN), lower root mean square differences between successive inter-beat intervals (RMSSD), as well as lower high-frequency power percentage and higher low-frequency power percentage at rest, indicating a higher sympathetic tone and/or lower parasympathetic/vagal tone in this population 37,38,39,40,41. IGD-related HRV abnormalities at rest were found to correlate with distressed personality total scores 38 and carving ratings 39, and thought to be linked to defective inhibitory control and reduced autonomic flexibility 42,43. A recent meta-analysis concluded that individuals with PIU have significantly different resting state parasympathetic activity from healthy controls 11. Consistent with the observations in those with alcohol addiction and gambling disorder 44,45, individuals with IGD also appeared to have higher HRV reactivity to game cues and during gameplay when compared to individuals without IGD. IGD individuals showed larger changes in low-frequency power/high-frequency power ratio (LF/HF) upon exposure to visual game cues 46. A significant decrease in high-frequency power (HF) during gameplay was observed in IGD individuals, but not in healthy controls 47. When playing a multiplayer online battle arena (MOBA) game, League of Legends (LOL), only individuals with IGD showed significant in-game HF decrease, the amplitude of which correlated negatively with IGD severity assessed by internet addiction test score 48,49. Game content and context are known to affect in-game HRV as well. Playing violent games induced greater changes in HRV compared to playing non-violent games 50,51. Playing a fighting game was associated with lower in-game RMSSD than playing puzzle games, and the players receiving threat appraisal instructions before gameplay showed significantly lower RMSSD than those received challenge appraisal instructions 52. Evidence also suggests that in-game HRV abnormalities observed in IGD individuals were situational, with different manifestations in different periods/phases of the game. For instance, some authors reported that IGD individuals had significant HF decreases in the early phase of the game when gameplay was still relatively easy and not complicated by real-time state of gaming 48,53, while others showed that HF in IGD individuals did not change in the early game phase, but instead decreased significantly when high attention was required, as well as in the last minutes of gameplay when game ending was approaching 49. Like in-game CNS activities, in-game ANS activities/abnormalities observed in these studies might be attributed to dynamic and complicated interactions between human factors of the player and mechanics of the game being played 54,55. Few previous studies have measured in-game HRV in habitual video gamers who are not formally diagnosed with IGD, or examined the factors influencing the in-game HRV in this population. In this study, real-time HR in habitual gamers (HGs) was recorded continuously with a wearable electroencephalogram (EEG) device when playing a round of LOL game under ergonomic and naturalistic conditions. The HR data were analyzed in two different ways. In the first analysis, short-term HRV in three 5-min time windows representing early (EG), middle (MG) and late (LG) phases of gameplay were computed and compared between the individuals at high risk of developing IGD and those at low risk. The aim of the first analysis is to test whether and how in-game HRV is affected by the risk of developing IGD. In the second analysis, multiple linear regression (MLR) was used to identify significant predictors of in-game HRV measured over the whole gameplay period (WG), aiming to test whether in-game HRV and ANS activities are affected by in-game behavioral performance and human characteristics of the player.
2.1. Participants
Forty-two right-handed male university students having at least 2 years of experience on LOL playing and, at the same time, having played more than 1000 LOL matches in the past were recruited. Written informed consent and demographic information (Table 1) were obtained from each participant before starting the experiments. The risk of developing IGD was assessed using the revised Chen Internet addiction scale (CIAS-R) 56. The skill level of the player in LOL was assessed using the ranking score provided by the official ranking system at the end of last LOL season (i.e., Season 2018). Self-reported hours of weekly LOL playing (HoWP) was used to assess the frequency of LOL playing in the past year. None of the participants had any neurological or psychiatric history, medical history affecting their ANS or sleep deprivation when they participated the study. All participants had normal or corrected normal vision, and were reported to have not used any neurological or psychiatric drugs in the 24 h before the experiment. The research was approved by Human Subjects Institutional Review Board of Wuhan Institute of Physics and Mathematics, Chinese Academy of Science (Protocol Number: WIPM20190303). Forty-two right-handed male university students having at least 2 years of experience on LOL playing and, at the same time, having played more than 1000 LOL matches in the past were recruited. Written informed consent and demographic information (Table 1) were obtained from each participant before starting the experiments. The risk of developing IGD was assessed using the revised Chen Internet addiction scale (CIAS-R) 56. The skill level of the player in LOL was assessed using the ranking score provided by the official ranking system at the end of last LOL season (i.e., Season 2018). Self-reported hours of weekly LOL playing (HoWP) was used to assess the frequency of LOL playing in the past year. None of the participants had any neurological or psychiatric history, medical history affecting their ANS or sleep deprivation when they participated the study. All participants had normal or corrected normal vision, and were reported to have not used any neurological or psychiatric drugs in the 24 h before the experiment. The research was approved by Human Subjects Institutional Review Board of Wuhan Institute of Physics and Mathematics, Chinese Academy of Science (Protocol Number: WIPM20190303).
2.2. Experimental Procedures
Each participant was asked to play a ranking-mode LOL match on a Dell computer (OptiPlex 3020SSF) with a 23.8-inch screen (DELL E2417H) in a sound-proof room, together with 9 anonymous online players selected automatically by the built-in matchmaking algorithm in the game. A chest strap equipped with a Polar H10 ECG sensor (Polar Electro Oy, Kempele, Finland) was used for HR recording at a sampling rate of 130 Hz 57. The HR data were recorded continuously for 1 min before the game onset and throughout the entire period of gameplay. No bystander was present in the room except the experimenters. Video data of the games were recorded at 15 frames/s using a built-in function provided by LOL. Each participant was asked to play a ranking-mode LOL match on a Dell computer (OptiPlex 3020SSF) with a 23.8-inch screen (DELL E2417H) in a sound-proof room, together with 9 anonymous online players selected automatically by the built-in matchmaking algorithm in the game. A chest strap equipped with a Polar H10 ECG sensor (Polar Electro Oy, Kempele, Finland) was used for HR recording at a sampling rate of 130 Hz 57. The HR data were recorded continuously for 1 min before the game onset and throughout the entire period of gameplay. No bystander was present in the room except the experimenters. Video data of the games were recorded at 15 frames/s using a built-in function provided by LOL.
2.3. HRV Measurement
The HR data were extracted from the Polar Best app (versions 2.3.1) and transformed into R-R intervals. The Kubios HRV Standard freeware (versions 3.3) was used to calculate HRV indices from the R-R interval time series 58. Square root of Baevsky’s stress index (STRESS) 59, three time domain indices (i.e., Mean HR, SDNN and RMSSD) and one nonlinear index (i.e., sample entropy (SampEn)) were calculated, as well as four frequency domain indices calculated with the autoregressive model, including low-frequency power (LF), HF, total power (TP) and LF/HF. The HR data were extracted from the Polar Best app (versions 2.3.1) and transformed into R-R intervals. The Kubios HRV Standard freeware (versions 3.3) was used to calculate HRV indices from the R-R interval time series 58. Square root of Baevsky’s stress index (STRESS) 59, three time domain indices (i.e., Mean HR, SDNN and RMSSD) and one nonlinear index (i.e., sample entropy (SampEn)) were calculated, as well as four frequency domain indices calculated with the autoregressive model, including low-frequency power (LF), HF, total power (TP) and LF/HF.
2.4. LOL Game
LOL is one of the most popular MOBA video games worldwide 60. It is known that playing MOBA games is associated with more time spent on video gaming and a higher endorsement of IGD symptoms 61. In each LOL game, 10 players summoned are divided into 2 ad hoc teams. Each player selects and controls his/her own avatar (or champion) to fight with the champions and no-player-controlled characters from the opponent team in a virtual game arena. To win the game, one team needs to destroy the base (or nexus) of the other team while preventing its own base being destroyed. A typical LOL game lasts 20–40 min and ends when the base of one team is destroyed or one team surrenders voluntarily.There is a seven-tier (i.e., Bronze, Silver, Gold, Platinum, Diamond, Master and Challenger) ranking system in LOL (Version 8.22), and within each tier there are five divisions, with five being the lowest in that tier and one being the highest. Game rank of the participants in this study ranged from Bronze to Master, with 5 in Bronze, 18 in Silver, 2 in Platinum, 16 in Diamond and 1 in Master. For statistical analyses, the categorical game ranks of the players were transformed into real number ranking scores using a linear coding system, with 1 representing tier Bronze and 6 representing tier Master. An increment of 0.2 was used to code each within-tier divisional promotion. For example, Division 5 in tier Sliver was coded as 2.0, and Division 3 in tier Diamond as 5.4.A typical LOL game can be divided roughly into EG, MG and LG phases, based on the interim goals and the gaming strategy the player would most likely adopt. Since there are no clear markers that can be used to tell the game phases apart, we defined them operationally. The 5 min immediately after game onset was taken as the EG phase, the 5 min around the midpoint of each game as the MG phase, and the last 5 min of the game as the LG phase. In-game behavioral parameters used for data analysis, including game length, number of slay (Nslay) and number of slain (Nslain), were read directly from the game log. LOL is one of the most popular MOBA video games worldwide 60. It is known that playing MOBA games is associated with more time spent on video gaming and a higher endorsement of IGD symptoms 61. In each LOL game, 10 players summoned are divided into 2 ad hoc teams. Each player selects and controls his/her own avatar (or champion) to fight with the champions and no-player-controlled characters from the opponent team in a virtual game arena. To win the game, one team needs to destroy the base (or nexus) of the other team while preventing its own base being destroyed. A typical LOL game lasts 20–40 min and ends when the base of one team is destroyed or one team surrenders voluntarily. There is a seven-tier (i.e., Bronze, Silver, Gold, Platinum, Diamond, Master and Challenger) ranking system in LOL (Version 8.22), and within each tier there are five divisions, with five being the lowest in that tier and one being the highest. Game rank of the participants in this study ranged from Bronze to Master, with 5 in Bronze, 18 in Silver, 2 in Platinum, 16 in Diamond and 1 in Master. For statistical analyses, the categorical game ranks of the players were transformed into real number ranking scores using a linear coding system, with 1 representing tier Bronze and 6 representing tier Master. An increment of 0.2 was used to code each within-tier divisional promotion. For example, Division 5 in tier Sliver was coded as 2.0, and Division 3 in tier Diamond as 5.4. A typical LOL game can be divided roughly into EG, MG and LG phases, based on the interim goals and the gaming strategy the player would most likely adopt. Since there are no clear markers that can be used to tell the game phases apart, we defined them operationally. The 5 min immediately after game onset was taken as the EG phase, the 5 min around the midpoint of each game as the MG phase, and the last 5 min of the game as the LG phase. In-game behavioral parameters used for data analysis, including game length, number of slay (Nslay) and number of slain (Nslain), were read directly from the game log.
2.5. Data Analysis
The HRV data were analyzed using two different methods. In the first analysis, the participants were divided into two groups according to their CIAS-R. The participants with a CIAS-R higher than 63 were considered to have a high risk of becoming addicted 56 and therefore assigned into the High-Risk group (n = 20), while those with a CIAS-R lower than 63 were assigned into the Low-Risk group (n = 22) (Table 1). An independent sample t-test or Mann–Whitney U test was used for statistical analysis of the inter-group differences in demographic and in-game behavioral parameters. Repeated measures analysis of covariance (RM-ANCOVA) was used to compare HRV measured in different game phases, with group as the between-subjects factor (High Risk vs. Low Risk), game phase as the within-subjects factor. All demographic parameters listed in Table 1, except CIAS-R, was used as covariates. Independent-sample and paired t-tests were used for post hoc between-subject and within-subject comparisons, respectively. False discovery rate (FDR) correction was applied to control type-I errors associated with multiple post hoc comparisons.In the second analysis, MLR was used to identify significant predictors for in-game HRV measured over WG (27 ± 5 min). All demographic and behavioral parameters listed in Table 1 were included in the regression model, except education and game length. Education had a high correlation with age (r = 0.794, p < 0.001), and game length showed significant correlations with both Nslay (r = 0.327, p = 0.034) and Nslain (r = 0.418, p = 0.008). Collinearity among the included parameters was assessed using the variation inflation factor (VIF). In cases where more than one significant predictor was identified, mediation and moderation analyses were used to examine the relationships between/among the significant predictors, controlling for the effects of all the other variables included in the MLR model.Normality of all data was verified with the Shapiro–Wilk tests. A p ≤ 0.05, corrected if applicable, was considered to be statistically significant. All statistical analyses were performed with the software package SPSS 20.0 (PC version). The HRV data were analyzed using two different methods. In the first analysis, the participants were divided into two groups according to their CIAS-R. The participants with a CIAS-R higher than 63 were considered to have a high risk of becoming addicted 56 and therefore assigned into the High-Risk group (n = 20), while those with a CIAS-R lower than 63 were assigned into the Low-Risk group (n = 22) (Table 1). An independent sample t-test or Mann–Whitney U test was used for statistical analysis of the inter-group differences in demographic and in-game behavioral parameters. Repeated measures analysis of covariance (RM-ANCOVA) was used to compare HRV measured in different game phases, with group as the between-subjects factor (High Risk vs. Low Risk), game phase as the within-subjects factor. All demographic parameters listed in Table 1, except CIAS-R, was used as covariates. Independent-sample and paired t-tests were used for post hoc between-subject and within-subject comparisons, respectively. False discovery rate (FDR) correction was applied to control type-I errors associated with multiple post hoc comparisons. In the second analysis, MLR was used to identify significant predictors for in-game HRV measured over WG (27 ± 5 min). All demographic and behavioral parameters listed in Table 1 were included in the regression model, except education and game length. Education had a high correlation with age (r = 0.794, p < 0.001), and game length showed significant correlations with both Nslay (r = 0.327, p = 0.034) and Nslain (r = 0.418, p = 0.008). Collinearity among the included parameters was assessed using the variation inflation factor (VIF). In cases where more than one significant predictor was identified, mediation and moderation analyses were used to examine the relationships between/among the significant predictors, controlling for the effects of all the other variables included in the MLR model. Normality of all data was verified with the Shapiro–Wilk tests. A p ≤ 0.05, corrected if applicable, was considered to be statistically significant. All statistical analyses were performed with the software package SPSS 20.0 (PC version). A total of 42 subjects were included in this study. Table 1 lists the demographic and in-game behavioral parameters of these subjects.
3.1. Analysis 1: Game Phase-Dependent Changes of In-Game HRV
The High-Risk group and Low-Risk group showed significant inter-group difference in CIAS-R (t = −10.865, p < 0.001), but not in any other demographic parameters (Table 1). Neither did The two groups show any statistically significant inter-group differences in game length, Nslay or Nslain (Table 1).Analyzing the data with RM-ANCOVA yielded statistically significant main effect of group for LF/HF (F(1,37) = 4.069, p = 0.050, η2 = 0.099, Figure 1a), but not for LF (F(1,37) = 0.303, p = 0.586, η2 = 0.008, Figure 1b), HF (F(1,37) = 2.519, p = 0.121, η2 = 0.064, Figure 1c) or any other HRV indices. A statistically significant main effect of game phase × group interaction was observed for STRESS (F(1,37) = 3.285, p = 0.043, η2 = 0.082). STRESS tended to be higher in EG than in MG (p = 0.073, FDR corrected) in the High-Risk group, while the Low-Risk Group did not show this trend (Figure 1d). No HRV index showed statistically significant main effects of game phase or game phase × covariant interactions, suggesting that statistically in-game HRV was not game phase-dependent. The High-Risk group and Low-Risk group showed significant inter-group difference in CIAS-R (t = −10.865, p < 0.001), but not in any other demographic parameters (Table 1). Neither did The two groups show any statistically significant inter-group differences in game length, Nslay or Nslain (Table 1). Analyzing the data with RM-ANCOVA yielded statistically significant main effect of group for LF/HF (F(1,37) = 4.069, p = 0.050, η2 = 0.099, Figure 1a), but not for LF (F(1,37) = 0.303, p = 0.586, η2 = 0.008, Figure 1b), HF (F(1,37) = 2.519, p = 0.121, η2 = 0.064, Figure 1c) or any other HRV indices. A statistically significant main effect of game phase × group interaction was observed for STRESS (F(1,37) = 3.285, p = 0.043, η2 = 0.082). STRESS tended to be higher in EG than in MG (p = 0.073, FDR corrected) in the High-Risk group, while the Low-Risk Group did not show this trend (Figure 1d). No HRV index showed statistically significant main effects of game phase or game phase × covariant interactions, suggesting that statistically in-game HRV was not game phase-dependent.
3.2. Analysis 2: Multiple Linear Regression of HRV Measured over WG
The results of enter MLR analysis of HRV measured over WG are listed in Table 2. The prediction models for HF (R2 = 0.392, F(6,35) = 3.761, p = 0.005), RMSSD (R2 = 0.390, F(6,35) = 3.731, p = 0.006) and STRESS (R2 = 0.303, F(6,35) = 2.534, p = 0.038) were statistically significant. HF was predicted by CIAS-R (β = −0.279, p = 0.040), ranking score (β = −0.472, p = 0.009) and Nslain (β = −0.532, p = 0.003). RMSSD was predicted by CIAS-R (β = −0.265, p = 0.047) and Nslain (β = −0.560, p = 0.002). STRESS was predicted by, and only by, Nslain (β = 0.519, p = 0.006). Without having statistically significant prediction models, LF/HF (β = 0.430, p = 0.008) was predicted by, and only by, CIAS-R. SDNN (β = −0.545, p = 0.005), LF (β = −0.517, p = 0.009) and TP (β = −0.562, p = 0.004) was predicted by, and only by, Nslain. All parameters included in the regression analysis had a VIF < 5, indicating that there was no significant collinearity among them in the regression analyses 62. Figure 2 plots LF/HF, LF, HF and STRESS against CIAS-R and Nslain, as well as the linear fits to the data.Neither the HF vs. CIAS-R correlation nor the RMSSD vs. CIAS-R correlation was moderated/mediated by NSlain, nor was the HF vs. CIAS-R correlation moderated/mediated by ranking score. However, the correlation between HF and ranking score was found to be partially mediated by Nslain, and vice versa. The mediating effects of Nslain (total effect = −1.950, p = 0.407; direct effect = −6.908, p = 0.010; indirect effect = 4.958, p < 0.001) and ranking score (total effect = −2.173, p = 0.056; direct effect = −4.175, p = 0.002; indirect effect = 2.002, p < 0.001) on each other manifested as a masking effect, given the opposite signs of the direct and indirect effects 63. Assessing pairwise correlations among the parameters entered into MLR yielded a negative correlation between Nslain and ranking score (r = −0.507, p = 0.001, Spearman’s correlation), which was also the only pairwise correlation that was statistically significant. The results of enter MLR analysis of HRV measured over WG are listed in Table 2. The prediction models for HF (R2 = 0.392, F(6,35) = 3.761, p = 0.005), RMSSD (R2 = 0.390, F(6,35) = 3.731, p = 0.006) and STRESS (R2 = 0.303, F(6,35) = 2.534, p = 0.038) were statistically significant. HF was predicted by CIAS-R (β = −0.279, p = 0.040), ranking score (β = −0.472, p = 0.009) and Nslain (β = −0.532, p = 0.003). RMSSD was predicted by CIAS-R (β = −0.265, p = 0.047) and Nslain (β = −0.560, p = 0.002). STRESS was predicted by, and only by, Nslain (β = 0.519, p = 0.006). Without having statistically significant prediction models, LF/HF (β = 0.430, p = 0.008) was predicted by, and only by, CIAS-R. SDNN (β = −0.545, p = 0.005), LF (β = −0.517, p = 0.009) and TP (β = −0.562, p = 0.004) was predicted by, and only by, Nslain. All parameters included in the regression analysis had a VIF < 5, indicating that there was no significant collinearity among them in the regression analyses 62. Figure 2 plots LF/HF, LF, HF and STRESS against CIAS-R and Nslain, as well as the linear fits to the data. Neither the HF vs. CIAS-R correlation nor the RMSSD vs. CIAS-R correlation was moderated/mediated by NSlain, nor was the HF vs. CIAS-R correlation moderated/mediated by ranking score. However, the correlation between HF and ranking score was found to be partially mediated by Nslain, and vice versa. The mediating effects of Nslain (total effect = −1.950, p = 0.407; direct effect = −6.908, p = 0.010; indirect effect = 4.958, p < 0.001) and ranking score (total effect = −2.173, p = 0.056; direct effect = −4.175, p = 0.002; indirect effect = 2.002, p < 0.001) on each other manifested as a masking effect, given the opposite signs of the direct and indirect effects 63. Assessing pairwise correlations among the parameters entered into MLR yielded a negative correlation between Nslain and ranking score (r = −0.507, p = 0.001, Spearman’s correlation), which was also the only pairwise correlation that was statistically significant. The current study aimed to characterize in-game HRV in male university student HGs when they played a round of an LOL game, and explore how the in-game HRV was modulated by human characteristics, especially CIAS-R, and in-game performance of the player. When dividing the players into the High-Risk and Low-Risk groups based on CIAS-R, it was shown that the High-Risk group had significantly higher LF/HF during gameplay, regardless of the game phase. The players in the High-Risk group, but not in the Low-Risk group, also tended to have higher STRESS in EG than in MG. Analyzing the data from all players together, a significant predictor for in-game HRV indices measured over WG was identified with enter MLR. LF/HF was found to be predicted by, and only by, CIAS-R. In comparison, HRV indices commonly used as indicators of sympathetic activity, such as STRESS, SDNN and TP, were predicted by, and only by, NSlain. HF and RMSSD, two HRV indices commonly used as indicators of parasympathetic activity, were predicted by both CIAS-R and Nslain, and additionally HF was also predicted by ranking score. HoWP had little influence on in-game HRV. The results of the two analyses demonstrated, convergently, that the players at high risk of developing IGD had higher LF/HF during gameplay (Figure 1a and Table 2). HF/LF is an HRV index frequently used to assess the balance between the sympathetic and parasympathetic branches of the ANS 59,64,65. Previous research has reported that individuals with IGD have higher LF/HF at rest compared to healthy controls 37,47. Interestingly, individuals with alcohol and methamphetamine dependence are also known to have higher LF/HF at rest, presumably reflecting a shifted autonomic balance towards a sympathetically dominated state 66 or cardiotoxic/neurotoxic autonomic dysbalance 67. The results obtained in this study are consistent with and expand upon these previous findings, by showing that the players at high risk of developing IGD (i.e., CIAS-R > 63), but yet to be officially diagnosed with IGD, had elevated LF/HF during online video game playing. Moreover, it was demonstrated that LF/HF was higher in the players at high risk of developing IGD in all three game phases, suggesting that this feature may not be less related to the task load and cognitive demand of gameplay, but rather a personal attribute of the player, which would manifest both at rest and during gameplay. Elevated in-game LF/HF in the players at high risk of developing IGD appeared to be driven mainly by reduced HF. In Analysis 1, HF appeared to be lower in the High-Risk group than in the Low-Risk group (Figure 1c), although the difference did not reach statistical significance. In Analysis 2, CIAS-R was identified to be a significant predictor of HF, but not of LF (Table 2). Like RMSSD, HF is a well-established indicator of parasympathetic (vagal) tone 64,68. In comparison, the interpretation of LF is more complicated. It is argued by some authors that LF is related to both sympathetic and parasympathetic activities 65,69. Both HF and RMSSD were negatively predicted by CIAS-R, suggesting that in-game parasympathetic activity was lower in the players at high risk of developing IGD. This observation is consistent with previous reports showing that only subjects with IGD, but not healthy controls, had significant HF decreases during gameplay compared to pre-game baseline 48,49. STRESS is sensitive to amplification of sympathetic tone and considered an HRV index reflecting the activity of sympathetic regulation gears 59. In Analysis 1, the High-Risk group showed higher STRESS in EG than in MG (Figure 1d). This observation is in line with previous reports showing that individuals with IGD have significant decreases in HF during the first 5 min of LOL playing 48,53. On the other hand, it seemingly contradicts the finding of Hong et al., who reported no significant HF changes in the first 5 min in the IGD subjects 49. The mechanisms underlying game phase-related sympathetic/parasympathetic activation in the players at high risk of developing IGD remain to be elucidated. Increased reactivity to game cues, such as anticipation and anxiety, might have given rise to elevated STRESS in EG in the players at high risk of developing IGD. Previous studies have demonstrated that exposure to visual game cues is sufficient to induce significant sympathetic activations in individuals with IGD, manifesting as increased respiratory rate and decreased standard deviation of HR 70. Upon exposure to game cues, individuals with IGD also exhibited higher activations in distributed brain regions in the fronto–striatal–limbic networks, which are known to be involved in planning, motivational encoding and reward processing 71,72,73. Attenuated impulse control might have contributed to enhanced reactivity of the CNS and ANS to game cues in these individuals 74,75,76. A number of HRV indices were found to be predicted significantly by Nslain, including both the indicators of parasympathetic (i.e., HF and RMSSD) activity and those of sympathetic (i.e., STRESS, SDNN and TP) activity. STRESS increases with sympathetic activation 59, and TP and SDNN decrease with sympathetic activation 77,78,79. Collectively, the results listed in Table 2 suggest that in-game sympathetic activity increases with NSlain, while in-game parasympathetic activity decrease with NSlain. Slain is generally considered as a negative event in LOL. The player showing a count of slain that is above the team average is often considered an easy pick and often targeted at by the opponent team deliberately. At the same time, he or she may face destructive criticism from the teammates and a risk of being reported as a feeder or griefer. Nslain represents the total number of times a player is slain in WG. Therefore, having a high Nslain, for most of the time, would mean the player has had poor performance and unpleasant experience in the game. Numerous studies have shown that task-related changes in HRV are modulated by physical/cognitive demands, subjectively perceived difficulty, mental stress and task performance 31,80,81,82. Consistently, these studies show that tasks with higher difficulty and heavier cognitive demand are in general associated with higher level of mental stress, augmented sympathetic activations and weakened parasympathetic activities 31,83,84. Our observations concerning the prediction of in-game HRV by NSlain are consistent with these previous findings, supporting the notion that the poorer the player’s performance during gameplay, the more stressed he/she may feel, consequently leading to enhanced sympathetic activities and reduced parasympathetic activities. While the in-game sympathetic activity was mainly determined how well the player performed during gameplay, the in-game parasympathetic (vagal) activity is modulated by both behavioral performance and personal attributes of the player, as evidenced by the prediction of RMSSD and HF by both NSlain and CIAS-R. These results suggest that the sympathetic and parasympathetic branches are likely regulated through dissociable psychophysiological pathways during online video game playing, with the parasympathetic branch more closely related to the propensity of developing IGD. Additionally, HF was negatively predicted by ranking score (Table 2). It should be noted that NSlain correlated negatively with ranking score as well, and the correlation between HF and ranking score was found to be partially mediated by NSlain, and vice versa. Both the direct effects and indirect effects of ranking score and NSlain on HF were statistically significant, albeit in opposite signs. This means that ranking score and NSlain can modulate HF significantly and independently, despite that the effects of the two tend to mask each other. In other words, the high-ranking players would show an in-game HF lower than that observed in the low-ranking players, when they have the same NSlain; and the players with a high NSlain would have an in-game HF lower than that observed in those with a low NSlain, when they have the same ranking score. The negative correlation between ranking score and in-game HF could have arisen from the game mechanics of LOL. The matchmaking algorithm of LOL summons only those with comparable gaming records and skill levels to play together in a game, and in principle this practice customizes the game difficulty for each participant. However, the gameplay intensity and cognitive load in absolute terms may, still, vary among the games played by the players at different ranks. In an MOBA game, the collective actions of all players influence how the game unfolds and how competitive it is. It therefore may be true that the high-ranking players need to be more immersed and use higher and more complicated cognitive exertions, such as extra vigilance, divided attention and multi-tasking, to achieve a favorable outcome, as the margins for lapses and errors are narrower for them than for those at lower ranks. It is known that increases in immersion (i.e., flow), cognitive demand and “adrenaline-rush” experience during video game playing give rise to the so-called “flow” state, which is characterized by parasympathetic withdrawal-associated arousal and a decrease in HF 85,86,87,88. Consistent with our observation, previous studies have demonstrated that expert neurointerventionalists have lower HF than non-experts when performing simulated neuroangiographies with the same workload 89, and familiarized chess players have lower HF than unfamiliarized chess players when playing chess in a strange computer environment 30. It should be noted that there are a number of reports in the literature showing that experts tend to have higher HF than non-experts at rest 90,91 and the individuals having a higher HF at rest often display superior performance in cognitive tasks, such as N-back task and Stroop task 92,93. High-performance chess players are shown to have higher RMSSD than low-performance chess players when playing chess at low- to medium-difficulty levels, but not at high-difficulty levels 31. These results highlight that task-related changes in parasympathetic activity are most likely modulated by the complex interactions among the expertise, task difficulty and contingency of behavioral performance, rather than expertise per se. There are several limitations that need to be addressed. Firstly, complementary measurements of physiological indices such as respiration rate, blood pressure and skin conductance are required to provide a more complete and comprehensive understanding on how in-game autonomic activities are affected by in-game behaviors and personal attributes of the player. For example, respiratory parameters are important determinants of respiratory sinus arrhythmia (RSA), which may affect LF and HF independent of changes in cardiac vagal tone. Decreased respiratory rate is usually accompanied with increased LF 94. Therefore, the possible contribution of altered respiratory rhythm to the relatively higher LF/HF observed in the players at high risk of developing IGD cannot be excluded, given the reports showing that these players tended to have a lower respiration rate and/or hold breath more often during VGP 95. Further studies are needed to clarify how changes of breath rhythm affect the measurement and interpretation of in-game HRV. Secondly, since baseline HRV was not measured in this study, it remained unclear to what extent in-game HRV was related to and influenced by resting state HRV. A, LOL game typically lasts 20–40 min. Our preliminary experiments showed that it is challenging for subjects to remain in a resting state for an extended period of time that is comparable to the actual gameplay (i.e., tens of minutes). Thirdly, the data were obtained from a relatively small and homogenous group of players who played a single game. Further research is required to investigate whether the observed results can be generalized to other player populations and games. Finally, the precision, accuracy and interpretation of HRV measurements are known to depend on the length of the time window during which the heart beats are recorded 79. HRV measurements at various timescales may capture different aspects of in-game autonomic changes and their associations with in-game behaviors and psychophysiological states 96. HRV measurements were used to detect changes in autonomic activities during online video game playing in male university student HGs. In-game LF/HF, an HRV indictor of autonomic balance, was found to be higher in the players at high risk of developing IGD, giving further support to the notion that this HRV index may potentially be used for IGD screening and/or diagnosis. Poor performance during gameplay was found to be linked to higher sympathetic activity and lower parasympathetic activity. The in-game performance also, in part, mediated the modulating effect of ranking score on HRV indicators of parasympathetic activity, including HF. Last but not least, the players at high risk of developing IGD tended to have higher STRESS in EG than MG, probably reflecting enhanced reactivity to game cues in this population. Together, the results of this study suggest that IGD-related autonomic dysregulation may have arisen from the complex interactions among personal attributes and in-game behavioral performance of the player, as well as factors embedded in the mechanics of the game being played. Conceptualization, K.L., X.Z. and H.L.; methodology, K.L. and N.W.; investigation, K.L., X.Z. and N.W.; writing—original draft preparation, K.L.; writing—review and editing, H.L.; visualization, K.L.; supervision, H.L.; funding acquisition, H.L. All authors have read and agreed to the published version of the manuscript. This work was supported by a grant from the National Natural Science Foundation of China (21790392). This study protocol was approved by the Human Subjects Institutional Review Board of Wuhan Institute of Physics and Mathematics, Chinese Academy of Science (WIPM20190303) (approved date 3 March 2019). All patients receive written information about the research project, benefits and risks of participation. They are informed that they can withdraw from the study at any time. Written informed consent was obtained prior to intake assessment. Informed consent was obtained from all participants involved in the study. The data presented in this study are available on request from the corresponding author. The data are not publicly available due to the fact that they constitute an excerpt of research in progress. The authors declare no conflict of interest. 378. Reed, G.M.; First, M.B.; Kogan, C.S.; Hyman, S.E.; Gureje, O.; Gaebel, W.; Maj, M.; Stein, D.J.; Maercker, A.; Tyrer, P.; et al. Innovations and changes in the ICD-11 classification of mental, behavioural and neurodevelopmental disorders. World Psychiatry2019, 18, 3–19. Google Scholar CrossRef PubMed 379. Ko, C.H.; Hsieh, T.J.; Wang, P.W.; Lin, W.C.; Yen, C.F.; Chen, C.S.; Yen, J.Y. Altered gray matter density and disrupted functional connectivity of the amygdala in adults with Internet gaming disorder. Prog. Neuro-Psychopharmacol. Biol. Psychiatry2015, 57, 185–192. Google Scholar CrossRef PubMed 380. Lin, X.; Dong, G.; Wang, Q.; Du, X. Abnormal gray matter and white matter volume in ‘internet gaming addicts’. Addict. Behav.2015, 40, 137–143. Google Scholar CrossRef PubMed 381. Jin, C.; Zhang, T.; Cai, C.; Bi, Y.; Li, Y.; Yu, D.; Zhang, M.; Yuan, K. Abnormal prefrontal cortex resting state functional connectivity and severity of internet gaming disorder. Brain Imaging Behav.2016, 10, 719–729. Google Scholar CrossRef PubMed 382. Yuan, K.; Qin, W.; Yu, D.; Bi, Y.; Xing, L.; Jin, C.; Tian, J. Core brain networks interactions and cognitive control in internet gaming disorder individuals in late adolescence/early adulthood. Brain Struct. Funct.2016, 221, 1427–1442. Google Scholar CrossRef PubMed 383. Zhang, J.T.; Yao, Y.W.; Li, C.S.; Zang, Y.F.; Shen, Z.J.; Liu, L.; Wang, L.J.; Liu, B.; Fang, X.Y. Altered resting-state functional connectivity of the insula in young adults with internet gaming disorder. Addict. Biol.2016, 21, 743–751. Google Scholar CrossRef PubMed 384. Chen, C.Y.; Yen, J.Y.; Wang, P.W.; Liu, G.C.; Yen, C.F.; Ko, C.H. Altered Functional Connectivity of the Insula and Nucleus Accumbens in Internet Gaming Disorder: A Resting State fMRI Study. Eur. Addict. Res.2016, 22, 192–200. Google Scholar CrossRef PubMed 385. Dong, G.; Shen, Y.; Huang, J.; Du, X. Impaired error-monitoring function in people with internet addiction disorder: An event-related fMRI study. Eur. Addict. Res.2013, 19, 269–275. Google Scholar CrossRef 386. Shin, Y.B.; Kim, H.; Kim, S.J.; Kim, J.J. A neural mechanism of the relationship between impulsivity and emotion dysregulation in patients with Internet gaming disorder. Addict. Biol.2021, 26, e12916. Google Scholar CrossRef 387. Wang, Y.; Hu, Y.; Xu, J.; Zhou, H.; Lin, X.; Du, X.; Dong, G. Dysfunctional prefrontal function is associated with impulsivity in people with internet gaming disorder during a delay discounting task. Front. Psychiatry2017, 8, 287. Google Scholar CrossRef 388. Cheng, Y.C.; Huang, Y.C.; Huang, W.L. Can heart rate variability be viewed as a biomarker of problematic internet use? a systematic review and meta-analysis. Appl. Psychophysiol. Biofeedback2023, 48, 1–10. Google Scholar CrossRef PubMed 389. Lu, D.W.; Wang, J.W.; Huang, A.C. Differentiation of internet addiction risk level based on autonomic nervous responses: The internet-addiction hypothesis of autonomic activity. Cyberpsychol. Behav. Soc. Netw.2010, 13, 371–378. Google Scholar CrossRef PubMed 390. Spada, M.M. An overview of problematic internet use. Addict. Behav.2014, 39, 3–6. Google Scholar CrossRef PubMed 391. de Zambotti, M.; Trinder, J.; Silvani, A.; Colrain, I.M.; Baker, F.C. Dynamic coupling between the central and autonomic nervous systems during sleep: A review. Neurosci. Biobehav. Rev.2018, 90, 84–103. Google Scholar CrossRef 392. Patron, E.; Mennella, R.; Messerotti Benvenuti, S.; Thayer, J.F. The frontal cortex is a heart-brake: Reduction in delta oscillations is associated with heart rate deceleration. NeuroImage2019, 188, 403–410. Google Scholar CrossRef PubMed 393. Thayer, J.F.; Hansen, A.L.; Saus-Rose, E.; Johnsen, B.H. Heart rate variability, prefrontal neural function, and cognitive performance: The neurovisceral integration perspective on self-regulation, adaptation, and health. Ann. Behav. Med.2009, 37, 141–153. Google Scholar CrossRef 394. Shaffer, F.; McCraty, R.; Zerr, C.L. A healthy heart is not a metronome: An integrative review of the heart’s anatomy and heart rate variability. Front. Psychol.2014, 5, 1040. Google Scholar CrossRef 395. Al, E.; Iliopoulos, F.; Forschack, N.; Nierhaus, T.; Grund, M.; Motyka, P.; Gaebler, M.; Nikulin, V.V.; Villringer, A. Heart-brain interactions shape somatosensory perception and evoked potentials. Proc. Natl. Acad. Sci. USA2020, 117, 10575–10584. Google Scholar CrossRef 396. Barber, A.D.; John, M.; DeRosse, P.; Birnbaum, M.L.; Lencz, T.; Malhotra, A.K. Parasympathetic arousal-related cortical activity is associated with attention during cognitive task performance. NeuroImage2020, 208, 116469. Google Scholar CrossRef 397. Candia-Rivera, D.; Catrambone, V.; Thayer, J.F.; Gentili, C.; Valenza, G. Cardiac sympathetic-vagal activity initiates a functional brain-body response to emotional arousal. Proc. Natl. Acad. Sci. USA2022, 119, e2119599119. Google Scholar CrossRef 398. Whitehurst, L.N.; Cellini, N.; McDevitt, E.A.; Duggan, K.A.; Mednick, S.C. Autonomic activity during sleep predicts memory consolidation in humans. Proc. Natl. Acad. Sci. USA2016, 113, 7272–7277. Google Scholar CrossRef PubMed 399. Underwood, E. A sense of self-communication between the brain and other organs shapes how we think, remember, and feel. Science2021, 372, 1142–1145. Google Scholar CrossRef PubMed 400. Thayer, J.F.; Ahs, F.; Fredrikson, M.; Sollers, J.J., 3rd; Wager, T.D. A meta-analysis of heart rate variability and neuroimaging studies: Implications for heart rate variability as a marker of stress and health. Neurosci. Biobehav. Rev.2012, 36, 747–756. Google Scholar CrossRef PubMed 401. Camm, A.J.; Malik, M.; Bigger, J.T.; Breithardt, G.; Cerutti, S.; Cohen, R.J.; Coumel, P.; Fallen, E.L.; Kennedy, H.L.; Kleiger, R. Heart rate variability. Standards of measurement, physiological interpretation, and clinical use. Eur. Heart J.1996, 17, 354–381. Google Scholar CrossRef 402. Zhang, X.; Long, K.; Wang, N.; Zhang, J.; Lei, H. Synchronous measurements of prefrontal activity and pulse rate variability during online video game playing with functional near-infrared spectroscopy. J. Innov. Opt. Health Sci.2023, 16, 1–15. Google Scholar CrossRef 403. Sammito, S.; Thielmann, B.; Seibt, R.; Klussmann, A.; Weippert, M.; Böckelmann, I. Guideline for the application of heart rate and heart rate variability in occupational medicine and occupational science. ASU Int.2015, 2015, 1–29. Google Scholar CrossRef 404. Byun, S.; Kim, A.Y.; Jang, E.H.; Kim, S.; Choi, K.W.; Yu, H.Y.; Jeon, H.J. Detection of major depressive disorder from linear and nonlinear heart rate variability features during mental task protocol. Comput. Biol. Med.2019, 112, 103381. Google Scholar CrossRef 405. Keary, T.A.; Hughes, J.W.; Palmieri, P.A. Women with posttraumatic stress disorder have larger decreases in heart rate variability during stress tasks. Int. J. Psychophysiol.2009, 73, 257–264. Google Scholar CrossRef 406. Yeo, M.; Lim, S.; Yoon, G. Analysis of biosignals during immersion in computer games. J. Med. Syst.2017, 42, 3. Google Scholar CrossRef 407. Fuentes-Garcia, J.P.; Pereira, T.; Castro, M.A.; Carvalho Santos, A.; Villafaina, S. Heart and brain responses to real versus simulated chess games in trained chess players: A quantitative EEG and HRV study. Int. J. Environ. Res. Public Health2019, 16, 5021. Google Scholar CrossRef 408. Fuentes-Garcia, J.P.; Villafaina, S.; Collado-Mateo, D.; de la Vega, R.; Olivares, P.R.; Clemente-Suarez, V.J. Differences between high vs. low performance chess players in heart rate variability during chess problems. Front. Psychol.2019, 10, 409. Google Scholar CrossRef PubMed 409. Thielmann, B.; Hartung, J.; Bockelmann, I. Objective assessment of mental stress in individuals with different levels of effort reward imbalance or overcommitment using heart rate variability: A systematic review. Syst. Rev.2022, 11, 48. Google Scholar CrossRef PubMed 410. Marin-Morales, J.; Higuera-Trujillo, J.L.; Guixeres, J.; Llinares, C.; Alcaniz, M.; Valenza, G. Heart rate variability analysis for the assessment of immersive emotional arousal using virtual reality: Comparing real and virtual scenarios. PLoS ONE2021, 16, e0254098. Google Scholar CrossRef PubMed 411. Goncalves, S.F.; Chaplin, T.M.; Lopez, R., Jr.; Regalario, I.M.; Niehaus, C.E.; McKnight, P.E.; Stults-Kolehmainen, M.; Sinha, R.; Ansell, E.B. High-frequency heart rate variability and emotion-driven impulse control difficulties during adolescence: Examining experienced and expressed negative emotion as moderators. J. Early Adolesc.2021, 41, 1151–1176. Google Scholar CrossRef PubMed 412. Sakuragi, S.; Sugiyama, Y. Effects of reward and punishment on task performance, mood and autonomic nervous function, and the interaction with personality. J. Physiol. Anthropol.2009, 28, 181–190. Google Scholar CrossRef PubMed 413. Moon, E.; Lee, S.H.; Kim, D.H.; Hwang, B. Comparative study of heart rate variability in patients with schizophrenia, bipolar disorder, post-traumatic stress disorder, or major depressive disorder. Clin. Psychopharmacol. Neurosci.2013, 11, 137–143. Google Scholar CrossRef PubMed 414. Lin, P.C.; Kuo, S.Y.; Lee, P.H.; Sheen, T.C.; Chen, S.R. Effects of internet addiction on heart rate variability in school-aged children. J. Cardiovasc. Nurs.2014, 29, 493–498. Google Scholar CrossRef PubMed 415. Kim, N.; Hughes, T.L.; Park, C.G.; Quinn, L.; Kong, I.D. Altered autonomic functions and distressed personality traits in male adolescents with internet gaming addiction. Cyberpsychol. Behav. Soc. Netw.2016, 19, 667–673. Google Scholar CrossRef 416. Moretta, T.; Buodo, G. Autonomic stress reactivity and craving in individuals with problematic internet use. PLoS ONE2018, 13, e0190951. Google Scholar CrossRef 417. Moretta, T.; Sarlo, M.; Buodo, G. Problematic internet use: The relationship between resting heart rate variability and emotional modulation of inhibitory control. Cyberpsychol. Behav. Soc. Netw.2019, 22, 500–507. Google Scholar CrossRef 418. Park, S.M.; Lee, J.Y.; Choi, A.R.; Kim, B.M.; Chung, S.J.; Park, M.; Kim, I.Y.; Park, J.; Choi, J.; Hong, S.J.; et al. Maladaptive neurovisceral interactions in patients with internet gaming disorder: A study of heart rate variability and functional neural connectivity using the graph theory approach. Addict. Biol.2020, 25, e12805. Google Scholar CrossRef PubMed 419. Friedman, B.H. An autonomic flexibility-neurovisceral integration model of anxiety and cardiac vagal tone. Biol. Psychol.2007, 74, 185–199. Google Scholar CrossRef PubMed 420. Kuiper, M.W.M.; Verhoeven, E.W.M.; Geurts, H.M. Heart rate variability predicts inhibitory control in adults with autism spectrum disorders. Biol. Psychol.2017, 128, 141–152. Google Scholar CrossRef PubMed 421. Hwang, S.; Martins, J.S.; Douglas, R.J.; Choi, J.J.; Sinha, R.; Seo, D. Irregular autonomic modulation predicts risky drinking and altered ventromedial prefrontal cortex response to stress in alcohol use disorder. Alcohol. Alcohol.2021, 57, 437–444. Google Scholar CrossRef PubMed 422. Blanchard, E.B.; Wulfert, E.; Freidenberg, B.M.; Malta, L.S. Psychophysiological assessment of compulsive gamblers’ arousal to gambling cues: A pilot study. Appl. Psychophysiol. Biofeedback2000, 25, 155–165. Google Scholar CrossRef PubMed 423. Hsieh, D.L.; Hsiao, T.C. Heart rate variability of internet gaming disorder addicts in emotional states. In Proceedings of the 2016 International Conference on Biomedical Engineering (BME-HUST), Hanoi, Vietnam, 5–6 October 2016; pp. 155–158. Google Scholar 424. Chang, J.S.; Kim, E.Y.; Jung, D.; Jeong, S.H.; Kim, Y.; Roh, M.S.; Ahn, Y.M.; Hahm, B.J. Altered cardiorespiratory coupling in young male adults with excessive online gaming. Biol. Psychol.2015, 110, 159–166. Google Scholar CrossRef PubMed 425. Lee, D.; Park, J.; Namkoong, K.; Hong, S.J.; Kim, I.Y.; Jung, Y.C. Diminished cognitive control in internet gaming disorder: A multimodal approach with magnetic resonance imaging and real-time heart rate variability. Prog. Neuro-Psychopharmacol. Biol. Psychiatry2021, 111, 110127. Google Scholar CrossRef 426. Hong, S.J.; Lee, D.; Park, J.; Namkoong, K.; Lee, J.; Jang, D.P.; Lee, J.E.; Jung, Y.C.; Kim, I.Y. Altered heart rate variability during gameplay in internet gaming disorder: The impact of situations during the game. Front. Psychiatry2018, 9, 429. Google Scholar CrossRef 427. Lin, T.C. Effects of gender and game type on autonomic nervous system physiological parameters in long-hour online game players. Cyberpsychol. Behav. Soc. Netw.2013, 16, 820–827. Google Scholar CrossRef 428. Ivarsson, M.; Anderson, M.; Akerstedt, T.; Lindblad, F. Playing a violent television game affects heart rate variability. Acta Paediatr.2009, 98, 166–172. Google Scholar CrossRef 429. Porter, A.M.; Goolkasian, P. Video games and stress: How stress appraisals and game content affect cardiovascular and emotion outcomes. Front. Psychol.2019, 10, 967. Google Scholar CrossRef PubMed 430. Lee, D.; Hong, S.J.; Jung, Y.C.; Park, J.; Kim, I.Y.; Namkoong, K. Altered heart rate variability during gaming in internet gaming disorder. Cyberpsychol. Behav. Soc. Netw.2018, 21, 259–267. Google Scholar CrossRef PubMed 431. Neubauer, A.C.; Fink, A. Intelligence and neural efficiency. Neurosci. Biobehav. Rev.2009, 33, 1004–1023. Google Scholar CrossRef PubMed 432. Filho, E.; Husselman, T.A.; Zugic, L.; Penna, E.; Taneva, N. Performance gains in an open skill video-game task: The role of neural efficiency and neural proficiency. Appl. Psychophysiol. Biofeedback2022, 47, 239–251. Google Scholar CrossRef PubMed 433. Ko, C.H.; Yen, J.Y.; Yen, C.F.; Chen, C.C.; Yen, C.N.; Chen, S.H. Screening for internet addiction: An empirical study on cut-off points for the chen internet addiction scale. Kaohsiung J. Med. Sci.2005, 21, 545–551. Google Scholar CrossRef PubMed 434. Gilgen-Ammann, R.; Schweizer, T.; Wyss, T. RR interval signal quality of a heart rate monitor and an ECG Holter at rest and during exercise. Eur. J. Appl. Physiol.2019, 119, 1525–1532. Google Scholar CrossRef PubMed 435. Tarvainen, M.P.; Niskanen, J.P.; Lipponen, J.A.; Ranta-Aho, P.O.; Karjalainen, P.A. Kubios HRV–heart rate variability analysis software. Comput. Methods Programs Biomed.2014, 113, 210–220. Google Scholar CrossRef 436. Baevsky, R.; Berseneva, A. Use KARDiVAR system for determination of the stress level and estimation of the body adaptability: Standards of measurements and physiological interpretation. Kardivar. TV2009, 1149, d557. Available online: https://pdfs.semanticscholar.org/74a2/92bfafca4fdf1149d557348800fcc1b0f33b.pdf (accessed on 25 December 2023). 437. Maher, B. Can a video game company tame toxic behaviour. Nature2016, 531, 568–571. Google Scholar CrossRef 438. Rehbein, F.; King, D.L.; Staudt, A.; Hayer, T.; Rumpf, H.-J. Contribution of game genre and structural game characteristics to the risk of problem gaming and gaming disorder: A aystematic review. Curr. Addic. Rep.2021, 8, 263–281. Google Scholar CrossRef 439. Akinwande, M.O.; Dikko, H.G.; Samson, A. Variance inflation factor: As a condition for the inclusion of suppressor variable(s) in regression analysis. Open J. Stat.2015, 05, 754–767. Google Scholar CrossRef 440. MacKinnon, D.P.; Krull, J.L.; Lockwood, C.M. Equivalence of the mediation, confounding and suppression effect. Prev. Sci.2000, 1, 173–181. Google Scholar CrossRef PubMed 441. Malliani, A.; Pagani, M.; Lombardi, F.; Cerutti, S. Cardiovascular neural regulation explored in the frequency domain. Circulation1991, 84, 482–492. Google Scholar CrossRef PubMed 442. Reyes del Paso, G.A.; Langewitz, W.; Mulder, L.J.; van Roon, A.; Duschek, S. The utility of low frequency heart rate variability as an index of sympathetic cardiac tone: A review with emphasis on a reanalysis of previous studies. Psychophysiology2013, 50, 477–487. Google Scholar CrossRef PubMed 443. Jochum, T.; Reinhard, M.; Boettger, M.K.; Piater, M.; Bar, K.J. Impaired cerebral autoregulation during acute alcohol withdrawal. Drug Alcohol Depend.2010, 110, 240–246. Google Scholar CrossRef PubMed 444. Henry, B.L.; Minassian, A.; Perry, W. Effect of methamphetamine dependence on heart rate variability. Addict. Biol.2012, 17, 648–658. Google Scholar CrossRef PubMed 445. Mietus, J.E.; Peng, C.K.; Henry, I.; Goldsmith, R.L.; Goldberger, A.L. The pNNx files: Re-examining a widely used heart rate variability measure. Heart2002, 88, 378–380. Google Scholar CrossRef PubMed 446. Watson, A.M.; Hood, S.G.; Ramchandra, R.; McAllen, R.M.; May, C.N. Increased cardiac sympathetic nerve activity in heart failure is not due to desensitization of the arterial baroreflex. Am. J. Physiol.-Heart C2007, 293, H798–H804. Google Scholar CrossRef 447. Kim, H.; Ha, J.; Chang, W.D.; Park, W.; Kim, L.; Im, C.H. Detection of craving for gaming in adolescents with internet gaming disorder using multimodal biosignals. Sensors2018, 18, 102. Google Scholar CrossRef 448. Liu, L.; Yip, S.W.; Zhang, J.T.; Wang, L.J.; Shen, Z.J.; Liu, B.; Ma, S.S.; Yao, Y.W.; Fang, X.Y. Activation of the ventral and dorsal striatum during cue reactivity in internet gaming disorder. Addict. Biol.2017, 22, 791–801. Google Scholar CrossRef 449. Zhang, J.T.; Yao, Y.W.; Potenza, M.N.; Xia, C.C.; Lan, J.; Liu, L.; Wang, L.J.; Liu, B.; Ma, S.S.; Fang, X.Y. Effects of craving behavioral intervention on neural substrates of cue-induced craving in internet gaming disorder. NeuroImage Clin.2016, 12, 591–599. Google Scholar CrossRef PubMed 450. Zhang, J.; Chen, S.; Jiang, Q.; Dong, H.; Zhao, Z.; Du, X.; Dong, G.-H. Disturbed craving regulation to gaming cues in internet gaming disorder: Implications for uncontrolled gaming behaviors. J. Psychiatr. Res.2021, 140, 250–259. Google Scholar CrossRef PubMed 451. Wang, Y.; Wu, L.; Wang, L.; Zhang, Y.; Du, X.; Dong, G. Impaired decision-making and impulse control in Internet gaming addicts: Evidence from the comparison with recreational internet game users. Addict. Biol.2017, 22, 1610–1621. Google Scholar CrossRef PubMed 452. Zha, R.; Tao, R.; Kong, Q.; Li, H.; Liu, Y.; Huang, R.; Wei, Z.; Hong, W.; Wang, Y.; Zhang, D.; et al. Impulse control differentiates internet gaming disorder from non-disordered but heavy internet gaming use: Evidence from multiple behavioral and multimodal neuroimaging data. Comput. Hum. Behav.2022, 130, 107184. Google Scholar CrossRef 453. Brand, M.; Rumpf, H.-J.; Demetrovics, Z.; King, D.L.; Potenza, M.N.; Wegmann, E. Gaming disorder is a disorder due to addictive behaviors: Evidence from behavioral and neuroscientific studies addressing cue reactivity and craving, executive functions, and decision-making. Curr. Addic. Rep.2019, 6, 296–302. Google Scholar CrossRef 454. Montano, N.; Porta, A.; Cogliati, C.; Costantino, G.; Tobaldini, E.; Casali, K.R.; Iellamo, F. Heart rate variability explored in the frequency domain: A tool to investigate the link between heart and behavior. Neurosci. Biobehav. Rev.2009, 33, 71–80. Google Scholar CrossRef PubMed 455. Stein, P.K.; Bosner, M.S.; Kleiger, R.E.; Conger, B.M. Heart rate variability: A measure of cardiac autonomic tone. Am. Heart J.1994, 127, 1376–1381. Google Scholar CrossRef PubMed 456. Shaffer, F.; Ginsberg, J.P. An overview of heart rate variability metrics and norms. Front. Public Health2017, 5, 258. Google Scholar CrossRef 457. Kim, H.G.; Cheon, E.J.; Bai, D.S.; Lee, Y.H.; Koo, B.H. Stress and heart rate variability: A meta-analysis and review of the literature. Psychiatry Investig.2018, 15, 235–245. Google Scholar CrossRef 458. Pulopulos, M.M.; Vanderhasselt, M.A.; De Raedt, R. Association between changes in heart rate variability during the anticipation of a stressful situation and the stress-induced cortisol response. Psychoneuroendocrinology2018, 94, 63–71. Google Scholar CrossRef 459. Luft, C.D.; Takase, E.; Darby, D. Heart rate variability and cognitive function: Effects of physical effort. Biol. Psychol.2009, 82, 164–168. Google Scholar CrossRef PubMed 460. Luque-Casado, A.; Perales, J.C.; Cárdenas, D.; Sanabria, D. Heart rate variability and cognitive processing: The autonomic response to task demands. Biol. Psychol.2016, 113, 83–90. Google Scholar CrossRef PubMed 461. Taelman, J.; Vandeput, S.; Gligorijević, I.; Spaepen, A.; Van Huffel, S. Time-frequency heart rate variability characteristics of young adults during physical, mental and combined stress in laboratory environment. In Proceedings of the 2011 Annual International Conference of the IEEE Engineering in Medicine and Biology Society, Boston, MA, USA, 30 August–3 September 2011; pp. 1973–1976. Google Scholar 462. Khoshnoud, S.; Alvarez Igarzabal, F.; Wittmann, M. Brain-heart interaction and the experience of flow while playing a video game. Front. Hum. Neurosci.2022, 16, 819834. Google Scholar CrossRef PubMed 463. Tozman, T.; Magdas, E.S.; MacDougall, H.G.; Vollmeyer, R. Understanding the psychophysiology of flow: A driving simulator experiment to investigate the relationship between flow and heart rate variability. Comput. Hum. Behav.2015, 52, 408–418. Google Scholar CrossRef 464. Kozhevnikov, M.; Li, Y.; Wong, S.; Obana, T.; Amihai, I. Do enhanced states exist? Boosting cognitive capacities through an action video-game. Cognition2018, 173, 93–105. Google Scholar CrossRef PubMed 465. Kozhevnikov, M.; Strasser, A.; Abdullah, M.A. Accessing the states of enhanced cognition in a gaming context: The importance of psychophysiological arousal. Cogn. Sci.2022, 46, e13106. Google Scholar CrossRef PubMed 466. Hinzmann, D.; Singer, M.; Schmelter, V.; Kreiser, K.; Gehling, K.; Strober, L.; Kirschke, J.S.; Schulz, C.M.; Schneider, F. Differences in beginner and expert neurointerventionalists” heart rate variability during simulated neuroangiographies. Interv. Neuroradiol.2022, 15910199221128439. Google Scholar CrossRef PubMed 467. Mazurek, K.; Koprowska, N.; Gajewski, J.; Zmijewski, P.; Skibniewski, F.; Różanowski, K. Parachuting training improves autonomic control of the heart in novice parachute jumpers. Biocybern. Biomed. Eng.2018, 38, 181–189. Google Scholar CrossRef 468. Beffara, B.; Bret, A.G.; Vermeulen, N.; Mermillod, M. Resting high frequency heart rate variability selectively predicts cooperative behavior. Physiol. Behav.2016, 164, 417–428. Google Scholar CrossRef 469. Magnon, V.; Vallet, G.T.; Benson, A.; Mermillod, M.; Chausse, P.; Lacroix, A.; Bouillon-Minois, J.B.; Dutheil, F. Does heart rate variability predict better executive functioning? a systematic review and meta-analysis. Cortex2022, 155, 218–236. Google Scholar CrossRef 470. Forte, G.; Favieri, F.; Casagrande, M. Heart Rate Variability and Cognitive Function: A Systematic Review. Front. Neurosci.2019, 13, 710. Google Scholar CrossRef PubMed 471. Song, H.-S.; Lehrer, P.M. The effects of specific respiratory rates on heart rate and heart rate variability. Appl. Psychophysiol. Biofeedback2003, 28, 13–23. Google Scholar CrossRef PubMed 472. Chiu, W.-Y.; Chen, L.-Y.; Chi, H.-M.; Hsiao, T.-C. Toward instantaneous frequency of respiration to investigate the risk of internet gaming disorder. In Proceedings of the 2021 43rd Annual International Conference of the IEEE Engineering in Medicine & Biology Society (EMBC), Virtual Conference, 1–5 November 2021; pp. 232–235. Google Scholar 473. Liegeois, R.; Li, J.; Kong, R.; Orban, C.; Van De Ville, D.; Ge, T.; Sabuncu, M.R.; Yeo, B.T.T. Resting brain dynamics at different timescales capture distinct aspects of human behavior. Nat. Commun.2019, 10, 2317. Google Scholar CrossRef PubMed
Share and Cite
MDPI and ACS StyleLong, K.; Zhang, X.; Wang, N.; Lei, H. Heart Rate Variability during Online Video Game Playing in Habitual Gamers: Effects of Internet Addiction Scale, Ranking Score and Gaming Performance. Brain Sci.2024, 14, 29. https://doi.org/10.3390/brainsci14010029 AMA StyleLong K, Zhang X, Wang N, Lei H. Heart Rate Variability during Online Video Game Playing in Habitual Gamers: Effects of Internet Addiction Scale, Ranking Score and Gaming Performance. Brain Sciences. 2024; 14(1):29. https://doi.org/10.3390/brainsci14010029 Chicago/Turabian StyleLong, Kehong, Xuzhe Zhang, Ningxin Wang, and Hao Lei. 2024. “Heart Rate Variability during Online Video Game Playing in Habitual Gamers: Effects of Internet Addiction Scale, Ranking Score and Gaming Performance” Brain Sciences 14, no. 1: 29. https://doi.org/10.3390/brainsci14010029 Note that from the first issue of 2016, this journal uses article numbers instead of page numbers. See further details here.
Article Metrics
NoNo
Article Access Statistics
For more information on the journal statistics, click here. Multiple requests from the same IP address are counted as one view. MDPI and ACS StyleLong, K.; Zhang, X.; Wang, N.; Lei, H. Heart Rate Variability during Online Video Game Playing in Habitual Gamers: Effects of Internet Addiction Scale, Ranking Score and Gaming Performance. Brain Sci.2024, 14, 29. https://doi.org/10.3390/brainsci14010029 AMA StyleLong K, Zhang X, Wang N, Lei H. Heart Rate Variability during Online Video Game Playing in Habitual Gamers: Effects of Internet Addiction Scale, Ranking Score and Gaming Performance. Brain Sciences. 2024; 14(1):29. https://doi.org/10.3390/brainsci14010029 Chicago/Turabian StyleLong, Kehong, Xuzhe Zhang, Ningxin Wang, and Hao Lei. 2024. “Heart Rate Variability during Online Video Game Playing in Habitual Gamers: Effects of Internet Addiction Scale, Ranking Score and Gaming Performance” Brain Sciences 14, no. 1: 29. https://doi.org/10.3390/brainsci14010029 Note that from the first issue of 2016, this journal uses article numbers instead of page numbers. See further details here.
Article Metrics
NoNo
Article Access Statistics
For more information on the journal statistics, click here. Multiple requests from the same IP address are counted as one view. here Multiple requests from the same IP address are counted as one view. Article Processing Charges Pay an Invoice Open Access Policy Contact MDPI Jobs at MDPI For Authors For Reviewers For Editors For Librarians For Publishers For Societies For Conference Organizers Sciforum MDPI Books Preprints.org Scilit SciProfiles Encyclopedia JAMS Proceedings Series LinkedIn Facebook Twitter
This site only collects related articles. Viewing the original, please copy and open the following link:Brain Sciences Free Full-Text Heart Rate Variability during Online Video Game Playing in Habitual Gamers: Effects of Internet Addiction Scale Ranking Score and Gaming Performance